Predictive analytics
In today’s constantly connected world where an increasingly large amount of data is collected and analyzed, predictive analytics plays a critical role in business. Predictive analysis is the discipline of using current and historical data to derive predictions about unknowns such as future trends and events. The modern digital enterprise can use predictive analytics in its business and IT to gain a competitive edge.
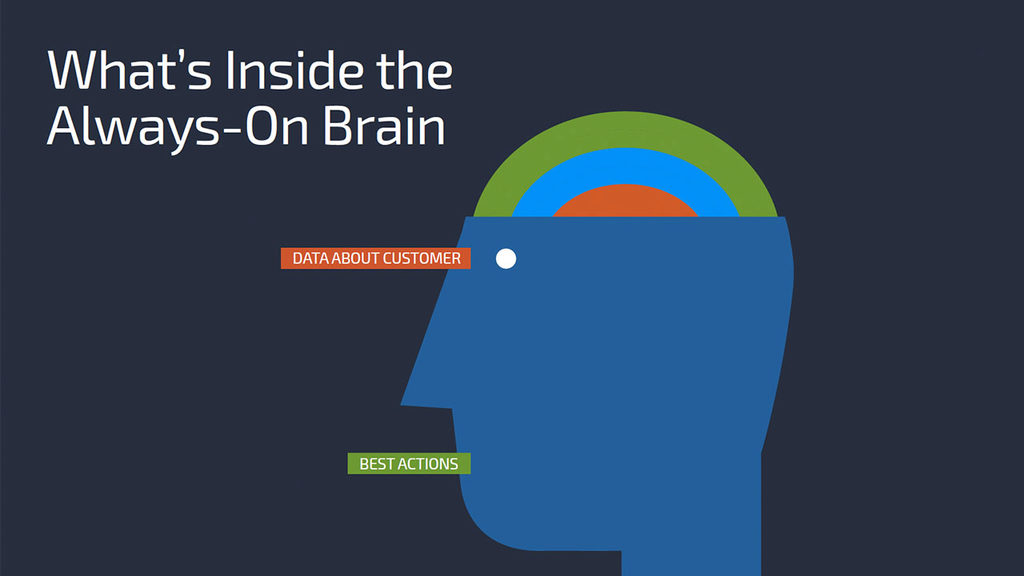
eBook
What’s inside the always-on brain?
Aspire to win over difficult customers efficiently and effectively with a personalized approach and predictive intelligence.
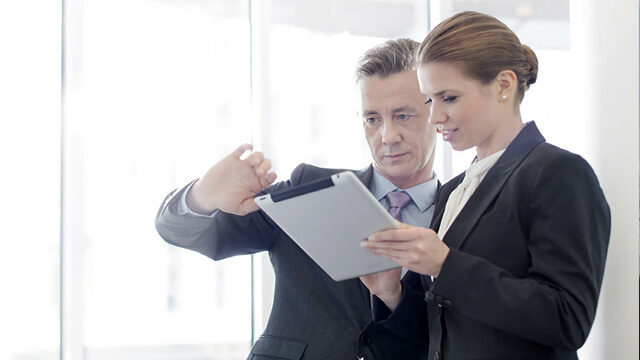
Data sheet
Pega predictive analytics director
To succeed, businesses need an easy, accurate and reliable way to create and incorporate predictive analytics and decisions into every process and interaction.
Build for Change: Real-time, Omni-channel AI
Pega AI delivers the right action at every customer touch by crunching millions of data points in real time.
How does predictive analytics work?
Predictive analytics is widely used today in many industries such as healthcare, life sciences, insurance, and finance. It returns the most value when the business strategy for its application is clearly identified. In practice, predictive analytics is most efficiently applied when integrated into business processes so that analytic insights and projections are used automatically by other systems such as a BPM system or CRM system.
Predictive analytics employ a combination of scientific methods and techniques such as the following:
- Data mining: Managing and analyzing large datasets, structured and unstructured, to identify patterns or relationships is the main function of data mining. Once identified, these patterns or relationships can be used to understand the behavior of the systems from which the data was collected.
- Modeling: In conjunction with data mining, statistical models can be built from collected data. Models are built based on the context of what needs to be predicted. Once built, new data can be input into the models to predict outcomes. For example, a sales organization can build a cross-selling model of existing customers to predict what other products they’d be most likely to buy from that company.
- Machine learning: Machine learning uses iterative methods and techniques to recognize patterns in large datasets and build models. Applications like recommendation engines for online shopping incorporate machine learning into their software. These engines make product recommendations based on predictions made from past customer buying and browsing behavior.
Pegasystems uses predictive analytics to increase customer value
Pega’s Next-Best-Action technology utilizes predictive analytics and real-time decisioning to recommend the interactions that will return the most value to your customers and your organization. Pega incorporates this technology in its CRM products for industries such as insurance (predictive analytics for insurance) and finance (predictive analytics in banking).
In today’s customer-driven business environment, brute force outbound marketing is not the best way to acquire new customers or retain existing ones. Customer engagement must be personalized in both inbound and outbound channels. Next-Best-Action creates relevant interactions with each of your customers to maximize cross-sell, up-sell, deep-sell, and retention opportunities. It employs predictive and adaptive models to determine customer interest and provides reusable marketing strategy templates, enabling your organization to design interactions quickly to adapt to changing customer needs.