Video
PegaWorld iNspire 2023: Harness the Power of Rapidly Evolving Tech to Drive Business Reinvention
The next wave of business transformation will create the foundations of a new reality – a shared reality that seamlessly converges the physical lives we’ve been leading with the digital lives that’s been rapidly expanding. In this session, we will explore the technology trends driving this new reality, and the steps enterprises will need to take to thrive in it, including strategies on digital identity, data transparency, and generative AI. Backed by Accenture research, these actionable insights will show how leaders can best use technology and get it right from the start. This is no longer a technical issue; it’s a business imperative.
Transcript:
- So before we get started, I've got a question. Anybody a hockey fan out there? Hockey. Hockey, you know who Wayne Gretzky was a while back. And I know not everybody's a hockey fan. So how about any soccer fans out there who know football, right, Lionel Messi, who's actually coming to my hometown in Miami, Florida, is now gonna be playing there. They both obviously were great, right? But what do they have in common? Anybody? Like what about them made them great? I know you just got back from lunch so . What's that? They're great players. They definitely are great players. Well, I asked the.
- Ability to think ahead.
- What's that?
- The ability to think ahead?
- I asked ChatGPT what makes them great players and what ChatGPT said is it's, you know, there are two of the greatest athletes in their respective sports. While they play different sports, there are some slammers in their style. But the main thing is both Gretzky and Messi are known for their vision, creativity, and ability to read the game. They can anticipate where the puck or ball will be, and make plays that others cannot see. And that's important. And what was interesting was I was thinking the same thing, right? And it was interesting that ChatGPT came up with that as well. But what's important, and this is really what we're going to talk about in this session today, is we're gonna talk about, we're gonna talk a little bit about setting context and where we are when it comes to technology, especially gen AI. We'll also touch a little bit on digital identity and digital transparency. But we'll spend a lot of time on Gen AI. But what's important is not necessarily about where things are today, but we want to have, and we have a set of experts here who are gonna talk about where things are going to be in the future. And the future is not that far away, right? Six months from now, 12 months from now, 18 months from now. That's really what the future that we're looking at. And so I have three experts in this space going from right to left. Many of you have worked in the Pega space know Shoel Perelman, and so Shoel is the VP for one-to-one customer engagement decisioning and AI for Pega. Next to Shoel is Lan Guan. And Lan is the Global Lead for data and AI in Accenture. And next to Lan is Christopher Yu. And Christopher Yu is the Head of Digital Transformation for US Bank. And really, really happy to have the three of them here today to go through this discussion as we jump into what we're seeing in the world today. And dive into it a little bit. But maybe what I'll start with is before we even jump into the topic of Gen AI I'll just ask Chris, 'cause with US Bank, maybe a lot of people are familiar with US Bank, if you can give a quick overview of that.
- Sure, so US Bank is the best bank that you've never heard of, as we like to say. The bank is headquartered in Minneapolis and maybe that sort of Minnesota nice DNA is part of the reason why we haven't been as self-promoting as some of our competition. But it's a bank which has a pretty extraordinary track record that has been built primarily on the retail banking side of the house and really focusing in on customer friendly experiences. And that's a tradition that we're trying to build on as we continue our digital transformation. I've been with the bank for about six years, since 2017, and have sort of grown my team as I was telling Shoel and Lan from a team of one to being a somewhat larger group that punches above its weight at the bank. So we're very excited. We use a variety of different marketing technologies in our stack, but we are very excited about the fact that we're piloting Pega Customer Decision Hub. So I'll probably be learning a little bit from some of you about what the best practices are, but we're figuring out how to orchestrate it and orchestrate it with our bleeding edge capabilities at the bank. And that's really what it's all about, so.
- Thanks Chris. No great background on the bank. And I think one thing that I always thought I think is really exciting about US Bank is that your ability to be nimble, right? And ability to be agile in how you transform, puts you in a great position. Let me start with maybe Lan and you know, in the keynote today, Kareem said November, 2022 was like a volcanic eruption. So maybe we can jump into that a little bit. What happened in the AI world in November, 2022?
- Sure, so David, I think that's a very good depiction of what happened with the launch of ChatGPT right? In November, 2022. So I think the, you know, I hope everyone in the room here got the chance to play with ChatGPT or, you know, similar applications. So me as a veteran, right, in the AI industry, we truly believe that we're at the AI's inflection moment. So what happened in November, 2022? I think there are two significant things that I want to call out. I think first and foremost, you know, the launch of a ChatGPT I think, you know, we believe that this is the first time, first time AI has actually, you know, started to make sense to general public. I think in the past, right? AI has always been considered as, you know, something that, you know, it's only managed by a small group of people, right? You know, people who's wearing, you know, white coat in the lab, you know, they do a lot of, you know, a lot of technical things, right? To build different kinds of AI solutions. I think that there's always a perception out there, right? I was telling David earlier, I think the personal side of the, you know, story I want to quickly share with everyone. So my first encounter with AI was actually about 30 years ago. I'm dating myself now, so at the age of 16, I built my first robot, you know, and I was living in China. So the robot was used to, you know, teach village kids in China, learn you know English to speak English. So as you can imagine, right at that point in time, the technology was very basic, was very clunky. But also I remember, you know, in order for me to start getting to the class to learn how to build robot, my parents have to, you know, basically do, you know, almost like spend all of their money, right? So it was very, very hard and it was only very small group of people were able to get access to AI. It's no longer the case. Okay? So I think the fact that AI is getting more democratized every consumer, right, can access AI, AI makes sense to them, to me that's fundamentally different. Which brings to the second point, I think, you know, because of that, lots of the business leaders also started pondering, right, what does this mean for business transformation, right? Within their organization. I can tell you, you know, over the last couple months, I probably have had hundreds of conversations, including everything that, you know, my team was doing, right? Talking to clients in, you know, in different industries, banking, insurance, you know, this is one of the number one topics that everyone wants to talk about. And I think, you know, it may be easy, you know, easier for you to think that, okay, some industry clients, like for example, banking, right? Insurance, you know, telco, they seem to be ahead of everyone. When you think about this topic, what actually struck me is a lot of, you know, traditional industry clients, you know, who never thought that they would be doing AI. They also approach us, they also raise hand, say, hey you know, what does, you know, this kind of technology mean to me? And, you know, this is the first time that I actually think that I can start embracing this technology. To me that's something that very, very different.
- What an amazing story, right? From building a robot at 16 years old to help villagers in China. And then your example of just where AI is now starting to be used in other industries and maybe 'cause we started going down the discussion of industry. I'll ask Chris to talk a little bit about what your seeing in banking and where you think this gen AI might play.
- Yeah, I think that's a great question. We at US Bank specifically are using gen AI in limited situations, primarily on our unauthenticated website to optimize our content. It's a tremendous tool when it comes to being able to not only engage customers in a way that they find comprehensible, which is, you know, a difficult challenge in financial services, but to figure out sort of which versions of the content will be most successful, really speak to the customer, really help to answer their questions and educate them when they need education about financial processes. So that's clearly one opportunity. I think there's been a lot of discussion about the opportunities in customer service and financial services is definitely an industry where we have a lot of high touch processes. Everything from essential support and customer notification all the way through to sales in some complex, you know, high touch sales situations. So there's lots of room for generative AI among all of those kinds of situations. I think the challenge will be really figuring out when and how to use it and when actually the enterprise is prepared to use it effectively. There's a great blog or podcast that just got released by the "New Yorker," where David Remnick, the editor of the "New Yorker," interviews Sam Altman, the CEO of Open AI, and I think Sam sort of punctures some of Mr. Remnick's myths about how AI is going to replace writers for example. And Altman said, you know, we're probably not at that point where the technology can truly replace good writers might be able to put a couple of bad writers out of work, but not really the good ones. But he said, I do see the opportunity for a lot of disruption and a lot of job loss in sort of customer support functions. And I think that is something that a lot of large enterprises are gonna have to struggle with. Are you you ready for that kind of wholesale change if it really comes at the velocity that we expect it to? And, you know, what are the opportunities to upscale and retrain some of those resources. I mean, I think at US Bank we are thinking about it primarily in terms of augmentation. And I think, you know, most companies are probably thinking about that, but the speed of the change may take our breaths away.
- Yeah. Thanks Chris. And you hit on a number of topics. And I'm gonna come back in a moment to we'll talk a little bit more about kind of the human and machine interaction and whether AI and gen AI is gonna replace people, but before I do that, Shoel maybe you could talk a little bit about the role that Pega is going to play in this space. I know we heard a little bit about that this morning on the keynotes, maybe to double check.
- Sure, absolutely. Yeah. I actually, I was gonna tell the story a little bit building on what you were just saying about how, you know, in Pega, we've had NLP technology in the product for, you know, the past 10 years or so. But it was a small group of people, it was used in very niche ways. Like we had GPT-2 embedded in there to be able to answer questions, but you had to ask them in the exact right way. And sometimes the answer wouldn't be understandable to a normal human right? And I think like, we all got hit by this, you know, this volcano that, oh wow, now it's actually really good enough for normal people to be able to use this. So what we really had to do is we had to step back and we had to think about like, well we're a platform company, right? So the first thing is all of our clients and our partners like Accenture, who build on top of it, are gonna be expect to be able to just use this technology in their applications. So the first thing we did is we built it and we made it just part of Pega platform. So there's a new rule like Kareem was talking about, where you can, you know, it stores the prompts and it's able to take the prompts, map them back into the application layer, but then we realize that's not enough, right? There are some obvious use cases that we need to bake into the application layer as well, because we obviously, you know, we're not just a platform company. We have applications like CDH, Customer Decision Hub, which I'm responsible for, and customer service. So even there, we see our role as thinking through of the 1,000 flowers that are blooming, picking like the 15 or 20 that we think will really be useful and investing the difficult work in making the prompts perfect, excellent. Such that real people can use them. So the reason I mentioned the 1,000 flowers blooming is like many companies, we did a hackathon in February, right? We said free for all engineers go crazy come up with your own ideas. And a lot of them were kind of ridiculous. And I think by looking through those, we started to see a pattern. And one of the patterns was that whenever we saw use cases that took what came back from a large language model and displayed it immediately to an end customer, meaning like one of our client's customers, we all sort of like shivered. And we realized like, no, that's not okay. And so from there, this principle of human in the loop became a core guiding principle that we're gonna look for use cases where we can ask gen AI to augment the intelligence, but there's always gonna be a human, an employee of our client who looks at that, approves it, gives it a thumbs up, and then starts serving it up to our customers.
- Oh, yeah. And that whole idea of human and machine is amazing, right? I mean we've been talking about that for a while, but now this takes it to the next level. I do wanna hit on a topic maybe to get a little geeky a bit right around, you know, we talked about this change in November and this change, what's different about this AI and Lan, maybe you could talk a bit about like, foundational models, like why is that important, right?
- Sure, sure. I think the, yes, so allowed me to go a little bit behind the scene, right? You know, just unveil a couple things that I believe why an engine like this, why AI system like this is truly disruptive. And, you know, Shoel and Chris, feel free to add your thoughts here. So as I mentioned before, I grew up building models, right? Statistical models, yeah. Machine learning models. A lot of them are actually, you know, being, you know, used into the, you know, in Pega systems and things like that, right? To basically do prediction to do forecasting. So I think someone like me, you know, who grew up building models, I think to me this is a paradigm shift, okay? Complete paradigm shift when I think about foundation models and what is the foundation models in this case is basically the AI system, right? Pre-trained by large research organization or tech companies using, you know, lots of, lots of, you know, public available internet data, right? The, you know, data from Wikipedia, data from, you know, you know, the YouTube, every single piece of videos, right? Data from Reddit, right? Data from, you know, common data sets, right? About books, about, you know, novels and things like that. So I think the point here is, you know, all this publicly available data is becoming the knowledge layer of this, you know, gigantic AI, and that's the first step, right? That's the pre-trained nature of the foundation models. What that means is, you know, then the lots of millions of organizations business right downstream will be able to leverage this foundation model as the basis, right? To do adaptation so that, you know, you can actually, you know, you know, adapt the model, do prompting that Shoel was talking about, or you can even go a little bit deeper, right? To do the fine tuning by bringing your own data. It could be the banks, you know, product tutorial data, could be your pricing data. Obviously all this needs to be done in a highly secure environment, right? To support all kinds of downstream tasks. I think this kinds of paradigm shift to me is truly disruptive because that means, you know, now research organization or Microsoft or you know, Google, AWS, all these platform partners, they can focus on building those foundational models, improving them while, you know, millions of organizations can really just focus on adaptation, focusing on thinking about what are the use cases that will bring the most benefits to their customers. I think this kind of bifurcation, you know almost like everybody specialize in things that you are really good at, you know, will actually help propel the overall progress of the society. That's something that, you know, I think it's a fundamental reason that we're so excited about this technology.
- I'll even add, like even within Pega we're seeing there's this split between like the people who are those NLP people who like really have a root, they understand how the models work, they're all about fine tuning and training, you know, domain specific understanding on top of those foundational models, right? But that's a small group of people who can do that. The vast majority of the people in Pega who are building, they're just using the, you know, the pre-trained models, but being very careful about finding the just the right content to feed into those models and telling it, do not hallucinate. If you don't know the answer, don't answer. Right? And we're actually seeing tremendous success from that. So it's actually, I think, gonna be very interesting to see, obviously, like most things it's probably gonna be a blend, right? Where these two will come together.
- Yeah, I'll just add to that, you know, I think you're touching on absolutely one of the most crucial aspects of getting it right when it comes to the uses and applications of AI which is, Shoel exactly as you and Lan have said, kind of a very small percentage of the people who work in this space who are really expert enough to truly create the methodologies and to really kind of build the models themselves. And then there are all of the end users and the contributors. And I think what that raises in a lot of ways are questions of governance. You want to democratize, you want to be as transparent as possible because that's the power of the model. That's how the model learns. And yet at the same time, you want to know that the ways in which the human agents are influencing the model are responsible, and they're done ethically, and they're done also with an eye towards the performance of the model in a way that makes sense. And all of those require nuance and they require governance. And that I think is something that we're struggling with at US Bank. I don't think we pretend to have a perfect recipe for it. And I think a lot of players are in the same situation.
- Yeah I mean great discussion, you could tell there's a lot of passion around that. I mean, but think about this for a moment. I mean that is a game changer. The fact that you have a foundational model. So essentially AI is remembering, right? We didn't have that before, but it's, maybe that's the wrong word to use, but it's sort of doing that, it's remembering where it is, and then based upon that foundation, you're adding trading data and it's starting to learn more, and you're building a new foundation on top of the foundation. And the ability to be able to leverage that going forward is huge. But let's maybe shift over, because it creates a huge opportunity. It also creates challenges. And I know, Chris, you talked a little bit about, you know, started getting into governance and maybe Lan commented on you know, how do we deal with the potential threat, right? And is it a threat?
- Yeah, sure I think that's exactly the reason that we, from Accenture's side, we believe that every business leader needs to take, you know, very thoughtful approach, okay? So meaning there are two parts of the coin, right? You know, it's because of the disruptive nature of the technology, this is not something that, you know, you can afford to be left behind, right? You know so do experimentation, right? Actually in Accenture way, this is the, you know, the approach that we are recommending for every single client. We even set up, you know, foundation model sandbox, right? With some of the enterprise readiness kind of layers right? Within the sandbox so that, you know, different industry clients can actually start experimenting, right? This kind of technology. But that's only one part. I think the second part is understand the risk, different kinds of risk and limitations, you know, with this emergent technology and things like, you know, I always joke about the responsible AI in the past, right? It's a little bit like nice to have, right? To different industry clients, you know, but nowadays it is actually become a necessity, okay? So I think a lot of organizations, regardless which industry you are in regulated or non-regulated, now you need to start implementing what we call responsible AI 2.0, okay? Lots of common guardrails, you know, you know, the governance, you know, controls things like fairness, bias, you know, explainability, transparency, things that matter, even in the, you know, you know, in the days without generative AI. Now you also need to think about adding new controls, right? Newer controls like language and toxicity, just because of the, you know, the generative in nature of this AI, also think about, you know, your model could be most accurate, right? Whatever tuning that you're doing. But then what if the contents, the responses that AI is generating, is not aligned to your company value, right? Also, I think the, you know, the need for inclusion and diversity even in your workforce, so that people who's training AI who's doing this kind of fine tuning, right? You know, they can actually bring in, you know, that very diverse opinions and skillset, right? In the AI training process. I think tho those are the just examples of the newer controls or cybersecurity, how can I forget about that, right? So all these other newer things that, frankly, a lot of my clients are still at the wake up moment to say, oh, okay, how do we do this, right? So that doesn't mean that you're not using the technology, bringing all this together, you know, take a very thoughtful approach. I think this is what really matters.
- Actually wanna build on your point about cybersecurity, because I think there's a risk that I'm starting to see come up, even though over the last few months, which is, you mentioned that the AI is just learning, right? And it's getting better and better, but I think that the fact that a model doesn't just learn unless it's being trained is being lost on a lot of people, right? I find I get into a conversation about, well I shouldn't possibly pass data to this model because it might repeat it to a different customer. And I have to get into a conversation about, no, unless you are actually paying for NVIDIA A-100's and you are really doing training, it's not going to learn anything from it. So I think there's also some, like, some knowledge awareness that we're gonna have to sort of raise about that.
- But that is a potential concern. Right? I mean I was thinking about you, Chris, and is there a concern that actually you start giving it the bank's data and all of a sudden it could recommend a different bank, right?
- Absolutely. Yeah. We would want to prevent that. But I mean, aside from the competitive threats, you know, I think Shoel you used the phrase, if I'm not mistaken, the human in the machine, right? And I love that idea, and I think it aligns perfectly with what Lan was saying about having the most accurate model in the world. But the importance being on how we're going about training the models. There was a really interesting article in the "New York Times," a little while ago about the use of AI in medicine and the fact that there were a bunch of hospital researchers who were working on an AI model to diagnose asthmatics. And this sort of touched me because I'm an asthmatic and it runs in my family. And they were talking about how they, this was at Duke actually, and they discovered after about a year's worth of work and crunching through the numbers, that they had a fantastic diagnostic tool in terms of the AI app itself. But the problem was that the data itself was inherently biased because the doctors in the Duke hospital system had been slower to diagnose asthma in Hispanic children than in the rest of the population. And that's a problem with treating asthmatics, because time is one of the factors with the efficacy of the treatment. So all of the data essentially was biased and wrong. So you can train the model and the model can be the best model in the world, but there's that human curatorial element of really being able to understand what does this research mean right? And being able to contextualize the data for the model itself, which is critical to getting it right. And that's where ethics is not just, you know, an empty buzzword. It's really about the power of the model. It's the power of the model in the real world and about delivering results.
- I think the governance of training is especially important, 'cause just like humans, if we hear something, somebody could tell you, forget what you just heard, but you can't really, right? And the models are the same way. Like once they learn something, it's very hard to get them to unlearn it.
- Yeah, no, I think that's a great call out. I think that's one example of not so obvious things that oftentimes in my role, I have to remind the, you know, the clients, right? So also I think just to build on this theme, what we are seeing also in the industry, because I talked to, you know, clients across different industries, right? Actually two patents, okay? Two patents that we also surface in the white paper point of view that we publish a couple weeks ago. You know, basically one patent is they're, you know, very large clients, right? They tend to be thinking about customized different ways of customizing foundation models, okay? And then more I would say, you know, other 70, 80% of the clients right now their mindset is on the consumption layer, which I think is exactly, you know, some of the capabilities that Pega is launching will be a great fit for right? So on the customization level, right? Very large organization, they started going down the path because of the sum of the limitations concerns that they were having, right? And also because of a generic nature of all these foundational models like GPT-4 or Google Palm, or the new models that are coming from AWS, right? They all generic model, right? So now all these clients are started asking, okay how do I make this kind of models, right? More industry specific. Like in telco's case, how do I bring in, you know, telco product information? How do I connect 5G, you know, you know, network, how do I connect my routers, right? This kind of product information, so into the model. So that model is becoming more industry specific, right? And also I think because of the concerns over, you know, not just the data security, also model ownership, right? Where does this data go into this shared generic model, right? So that's another reason. So we're seeing that's one pattern, but more and more clients are, you know, they start embracing, right, you know, generative AI because of the capabilities, like, you know, Shoel can talk about, right? You know, available on the platform layer, right? So that they can just go in there, they don't need to bring in their own data, right? All this generative AI capabilities can actually be launched on the application level, right? Yes it's limited to the workflow, but actually it's, you know, what we consider as a low hanging fruits, right? For lots of organization to start using generative AI. So this is the two patterns that we're seeing.
- Yeah, and I think the idea there is to make it as simple, like people, they think ChatGPT is magic because it just works. That's kind of the way we think about it in the platform is to just be there. You don't have to be an expert. It just works.
- Yeah, let me ask, because we're talking really about, disruption, right? And we have the three of you here that actually in a way are representing three different industries, right? The software industry, a consulting industry, and the banking industry. And I'd like to get a perspective, again, as we talked about at the beginning, this is trying to look forward thinking about what may happen a year from now. But how do you see this? Maybe I'll start Shoel with yourself. How do you see this disrupting your industry, right? The low code software?
- Well, I think that, you know, a lot of the last six months have been about calling a GPT model and getting a response back, right? Which is great. We have wonderful use cases for that. But I think where this is going next is agents. Being able to have an agent that you ask a higher level goal of, and it is able to figure out which tools does it need to use in conjunction with each other to carry out that goal. So one of the applications for this that we're thinking about in our own product, Alan talked about it on stage is, it's not enough to just be able to answer questions about how do I do a certain thing in the product. It should also be able to look inside the application, understand what you've done so far, and offer to do the thing you asked for you in your application. And I think that also has a lot of implications for education and how people learn about what the product does. It's not just gonna be about sitting down and like sitting through a training course. It's gonna be in context of what I'm doing right now, teach me the thing that I need to know no more, no less. So I think that's one of the big areas that will change in software.
- So I think from our perspective, I think the disruption will come in two different ways in our business model. I think first and foremost, we believe that this is actually the next couple years, right? Is gonna bring actually new business, new growth opportunities for companies like Accenture just simply because this kind of demand is overwhelmingly high. And this is not the technology's come and go, this is not the hype. We make that very, very clear. That's our posture. You know, we believe that Accenture is playing a very different role, right? Very crucial role to help all these organizations business to, you know, truly start embracing, you know, generative AI, not just embracing the technology also because of, you know, other surrounding impact that each business would be facing, like for example, workforce impact, right? You know, Chris talked a little bit about the, you know, job market impact from, you know, his banking perspective, right? We're also seeing, you know, based on our research overwhelmingly you know, high impact right? Across different industry, again, we did not say this is the, you know, just means the job will be lost, right? Overall, we believe that this is a positive sum gain, right? In our research report, we actually indicated about 40%. So 40% of the total working hours globally is gonna get impacted by generative AI. And then we did very detailed analysis, right? Task by task, profession by profession, how much of each will be actually automated? How much of this will be augmented, right? And what's the role of a copilot personal assistant, right? To each of us? So I think that's one reason that, you know, even we a technology company now we believe that this is not just a technology conversation, this kind of, you know, complexity, many business face will bring, you know, new growth opportunities for us and also for our clients. And not to mention other things like changing management. You know, the many, many questions c-suite clients are asking, how do I think about operating model? What are the future skillset of my future talents, right? So I think those are all very, very common and you know, across the board. And I think the second disruption is actually we are actually doing generative AI in, you know, to ourself, right? There are many examples because again, because of the growth opportunities, we're also in the process of making our, you know, our work more efficient, right? You know, embedding generative AI into everything we do, corporate functions, service delivery. So I'm very proud of the team that is behind this.
- Awesome, so I'll build a little bit on some of what you've been saying, Lan. I mean I look at the disruptive opportunities from the perspective of a marketer, which is what I am by training. And in terms of the marketing area at US Bank, you know, there's some tremendously wildly positive disruption that's possible. We do think it's going to accelerate the way that we execute our marketing. We do think it's going to allow us to scale tremendously in terms of personalization and really be able to begin to cultivate those one-to-one relationships with the customer that seem almost mythical when we talk about them, but are now becoming realizable because of the sophistication of the technology and the sophistication of the intelligence. I think in the short term, that's gonna change the mechanics of how our teams produce marketing. It's going to go from labor anywhere from three weeks to three months to get a marketing campaign out the door to starting to be able to execute in hours to minutes. And really being able to launch, you know, an innumerable number of messages and calls to action and experiences that engage the customer and deploy them in a way that really speaks to their business needs and how they're trying to answer their own questions as consumers. But I think as we go further along in that evolutionary path, it's going to become quite interesting because I think there's the potential, at least from a marketing perspective, of a fundamental change in the way that we think about marketing as a discipline if you will, and as a business process, as a driver of business growth. And that is that certainly at US Bank, and I think talking to some of my peers here at Pega, within the banking industry in general, most of our management teams tend to look at marketing as a great value add. And when it starts to succeed, that's the opportunity for management to get in there and sort of micromanage a little bit more heavily what's going on, and to start actually pushing the buttons and pulling the levers and saying, no, no, no, we need more of this product and you know, this is where we should double down. And really almost wanting to, you know, top down manage at the executive level, the on the ground results with the customers, and really almost being able to reach from the boardroom into the customer's lives. And that gets a little bit insane very quickly when you think about it as an empirical driver of growth. And I think the power of the model that is already available in some respects in CDH and is going to become increasingly available in tools like CDH. Is that you can flip it over now into true pure performance driven marketing, and you can begin to show the boardroom. I mean, this is one of the things that's fascinating for us looking at scenario planner, is the ability to actually see the consequences of certain decisions and the ability to see, well if we just buy Fiat say we're going to grow this part of the business, that could be a tremendously successful strategy, or it could just be a pipe dream. And that is disruptive in its own way and in a way that I think a lot of companies aren't, they don't really have a full handle on yet.
- Yeah. I love the example of a hyper personalization. I think the, just to build on what Chris was talking about, I think, you know, in this case, the power of generative AI, not only, you know, is giving this kind of hyper personalization power to marketers also could potentially to end users, right? End customers. What do I mean by that? One client I'm actually working with, it's not in banking, raising hotel, hospitality industry. I think what they are doing is such a great example, again, of democratizing AI in hyper personalization space, right? So in that case, they actually have in addition to their hotel properties, they also have home villas, right? Private homes and things like that. So in this case, they're actually creating, you know, generative AI applications on their platforms such that all this homeowners right now will be able to, you know, create stunning right property, you know, pictures and create hyper-personalized, you know, images of the, you know, rooms that they are listing, right? Things like that. So they're actually making that capability available to the end users. Something that they told us used to cost $20,000, right? For each of the home, right? It's impossible unaffordable, right? For a lot of homeowners, now they're actually making that available on the platform. And you can imagine the, you know, better customer experience impact, right? Revenue growth impact. I just thought that's a, you know, just a different stories of the hyper personalization that I really like.
- Takes citizen development to a new level, right? I do wanna, I know we have a few minutes left maybe to pause for a moment and any questions that folks will have while we have our three experts up here? Yeah, we're gonna have to give you one of the mics.
- Which solution is Accenture? We are currently offering in context of Pega and generative AI, so some components or whatever. So because I'm deeply in Pega space, yeah. And it's of course interesting how how we match this. What we have currently. Thank you.
- I think you're asking like what can you do as a consultant to be able to take advantage of this new technology with Pega quickly? Yeah I think the simplest answer is there's a rule called Connect Generative AI coming in Infinity 23. And as a, if you're familiar with how Pega platform works, you'll be able to give it a prompt. You'll be able to pass data from your case in, and then it will take the response back. So you can have like a creative response, right? Where you just maybe use it, or you could have a very prescriptive response that would come back as a structured list and that becomes parsed and made available in the rest of your case. Yeah. So day one, the first one. The ones that we have the most experience with so far just internally have been GPT-35 Turbo and GPT-4. And yeah, we get the message from Microsoft, they're saying like, cool it with Da Vinci, use GPT-35 Turbo. So those are sort of the first two outta the gate, but we are absolutely playing with PaLM 2. We've found it doesn't quite respond in the same way to very strict instruction following which we need for these things, but they're sort of neck and neck.
- Shoel, quick question. So when you talk about Infinity 23, right? Are you talking about version 89 or is it 90?
- We are moving away from that version numbering scheme with the 8.4, 8.5. And so 23 is the name for the version after 8.8.
- Thank You.
- Yes, and if you're a technical person, you look deep down there, you will see 8.9, you know, 8.23 in some place, right? 'Cause we had to pick a number.
- Okay, it's a bit of a general question, so one of the things I've been discussing and talking about is this patent infringement, which is one of the things that's really powerful about gen AI is that it learns a lot when you feed it lots of data. So if you're a bank and you are saying a fraud process, and all the banks feed all the data in together to say, to detect fraud, then you have a really powerful model. But at the same time, the banks gonna go, why would I share my data with anybody else? And so how do you see that happening? Because the more you share the better the models. But how are you gonna then encourage competing competitors to share data? How do you think that's gonna work.
- It's a fascinating question. I'll just add that I had this conversation last night with a customer in the oil and gas industry, and I was expecting him to say, 'cause we were having very much the same discussion, and he actually surprised me. He said, you know, we don't really compete directly with our competitors. They have their oil fields, we have ours. And, you know, if we're able to train models up that are able to do a better job, like we kind of both win. Haven't taken them up on that offer yet, but yeah, I was surprised.
- I'll jump into it. My thoughts as well. I do think there are going to be areas like fighting fraud where you will see a lot of, you know, what are normally traditional competitors forming syndicates if you will, that will share their information specifically for purposes that benefit the industry as a whole. I mean I think we're starting to see some of those opportunities emerge in the industry when it comes to essentially opportunities for identity management. Because with the death of cookies and device IDs, the ability to sort of partner and to share your information on a kind of a second party basis is becoming more interesting and more powerful to a lot of companies. And I think we're going to start to see a lot of traditional competitors lay down their swords, as it were, you know because they'll all benefit from AI at scale when it comes to security and privacy.
- Building on that, if banks transparently share that, then it becomes kind of semi-public. How do you prevent criminal elements to make use of the same model to actually well circumvent the same very model that every bank uses, I mean.
- Well now you're asking for the secret of, you know, I think, you know, being a chief security officer and you know, all the rest of it, I can't pretend to know all of the wizardry that goes into that. And I think that's really at the heart of a lot of what's emerging in the industry around, you know, identifying fraudulent behavior and criminal behavior is that it is going to become an arms race and you're going to see that, you know, the sectors that are interested in sort of the criminal behavior and perpetuating it, they're gonna have to be very well funded, you know, and they're gonna have to be very technically sophisticated and some of them are, you know, so it's going to become increasingly an arms race between those types of parties and, you know, the industries that wanna pool their resources and that want to wanna defend, you know, at the highest standards as it were what the technology will allow.
- It's definitely gonna keep the security industry in business, right?
- Yeah. It'll be very lucrative for somebody.
- Well see, one of the interesting use cases that we've heard about recently to this point about data privacy is using models to ensure that there's a layer of governance to make sure that no PII data gets passed to a model. So it's sort of like having one model that maybe is more trusted guard another model
- It leads to another question Shoel. I mean, about, one thing that Pega's AI has always been good at is you're able to, you know, flip a transparency button and now as you start to bring gen AI which by definition is not transparent right? How are you gonna balance that going forward in the product?
- Yeah, I think it's all about, you know, where we use it. It's about, you know, obviously as the CDH guy, Customer Decision Hub, we invest a lot in explainability of decisions. So one of the places where we're using generative AI is actually being able to have a discussion with the person who has to understand why the decision is made and be able to explain it to them in language that they'll understand. But I think the models themselves, I think we're a ways off from seeing, just like a human brain. We can't really like look inside a brain and see the, you know, how the neurons have lined up. You could tell us, but I don't think, I haven't seen any research papers about being able to do that with generative AI models. So I think we're gonna have to be very careful, you know, at least for the time being on what we trust them with.
- Yep, that's true, and I think that's one thing that we believe will happen on the skillset side, right? The importance of this making decision will never go away, right? That's just the part of the business, you know, part of the business, right? But we are noticing that the need for something, not data scientists, right? We have lots of data scientists, right? The need for decision engineers, right? So this will be a new role, just like prompt engineers, right? Which I think, you know, a lot of people have been getting familiar with, right? The decision engineers who can take the insights, right? The knowledge from this kind of pre-trained foundational models so that you're not starting from the scratch but then, you know, feed them into this kind of optimization engine, orchestration engine, right? To turn them into, you know, combined with other business acumen, right? Actually turn them into, you know, rapid decisions. I think that's something that we think that will be, you know, pretty common and will be a new trend. So decision engineers, this is something, a very hot career that people needs to be thinking about.
- We have probably in this very room, we have people whose title is lead decision architect, and this is the sort of thing they have to grapple with is how do you balance the models with the hard filtering rules.
- Great. Well, I think we are pretty much gonna wrap up. I think we got about a few seconds left. I do want to thank our guests Shoel and Lon and Chris for coming up, just a fantastic discussion. It's been videotaped as well. So we will be able to share that I think soon. So if you think about what you said . I probably should have said that at the beginning, right? But thanks everyone for the questions as well.
Tags
Related Resources
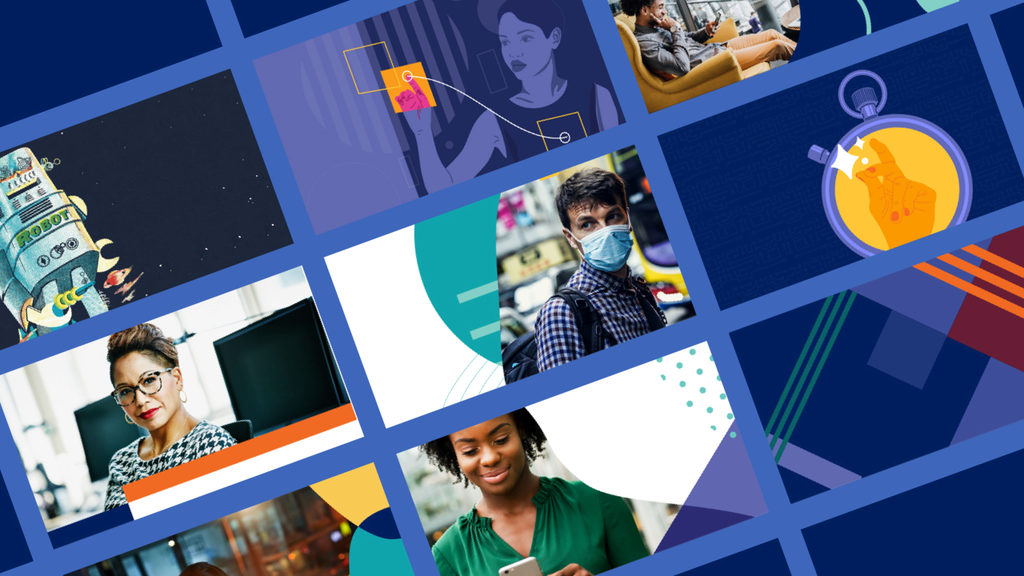
Recommended research & insights
See what’s new, what’s next, and what’s trending right now.
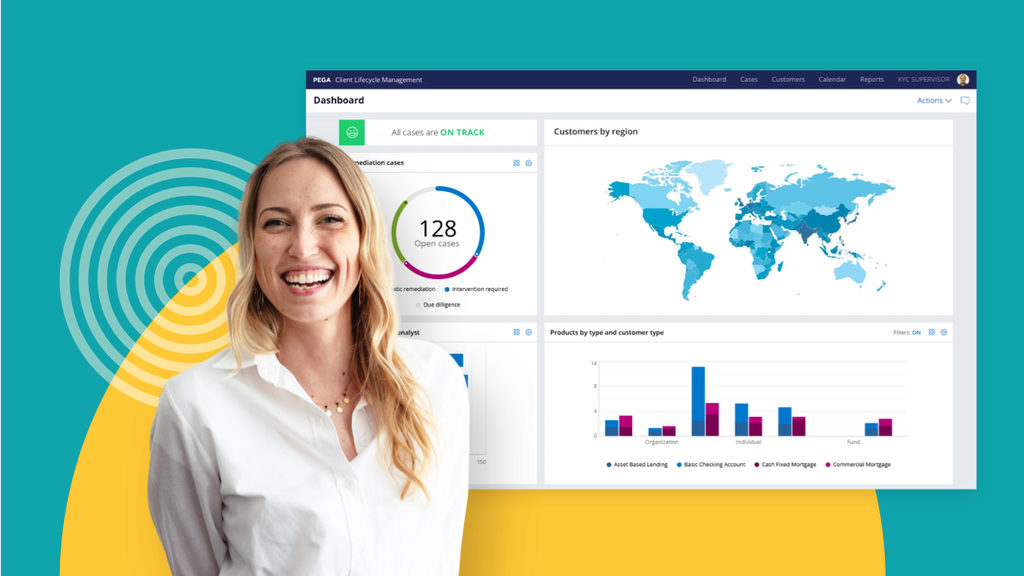
Why Pega?
Uniquely powerful software isn’t the only thing that sets us apart.