Video
How Knowledge Buddy works
Pega CTO Don Schuerman steps to a whiteboard to explain how Knowledge Buddy uses Retrieval Augmented Generation, or RAG, to turn your enterprise content into reliable answers powered by Pega GenAI. RAG minimizes the risks associated with GenAI by ensuring answers can be traced to source content, preventing hallucination, and keeping information up to date.
Transcript:
Hi, I am Don Schuerman. I'm here at the whiteboard today to talk a little bit about Pega's Knowledge Buddy product. Now, Knowledge Buddy, if you are not familiar with it, uses generative AI to synthesize the right answers from your own proprietary enterprise knowledge base. And it does that using a capability or a pattern called retrieval augmented generation, or RAG. And RAG is a really powerful technique because it addresses what are three challenges when you're using generative AI at the enterprise level.
The first challenge is you need traceability if you're gonna answer questions at the enterprise level. You know, if you ask ChatGPT for a burrito recipe, it'll give you a really good burrito recipe. It just can't tell you where it found that burrito recipe, which is fine for burritos, but probably not fine if you're trying to figure out how to process a claim or handle a payment exception.
The second challenge at the enterprise is you really can't handle hallucinations. And if you play with ChatGPT long enough, you know that every once in a while, if you ask it something it doesn't know, it very confidently makes up an answer. And we don't want that when we're operating at the enterprise level.
Third, we need to ensure at the enterprise level that our content is always up to date. Unfortunately, you know, GPT-3.5 was trained in 2021, GPT-4 was trained in 22/23. And you don't wanna have to retrain or fine tune a model, which can cost millions if not tens of millions of dollars every time you have new content. So, you need way to ensure that that content stays timely. RAG addresses all of this.
So, how does it work? Well, the first thing you do is you take all of your enterprise content, right, so that could be documentation, that could be how-to manuals, that could be marketing content. You know, at Pega we've used Knowledge Buddy on our Pega documentation, we used it internally for sales and marketing content. And you use AI to go through a process called embeddings where you basically pull out the little chunks of content from all of these documents. But not only you pulling out the chunks of content, you're understanding the semantic relationships between them. So, you understand that tortilla is related to wraps and wrapping a burrito, even though the word tortilla and wrap have no direct kind of semantic relationship. And you take those chunks of content and you put them into a special kind of database called a vector database. And a Vector database is really good at storing and keeping those semantic relationships. And we do that for you with Knowledge Buddy. Knowledge Buddy does all of this work for you automatically.
So, when you have Knowledge Buddy and your users or your customers come in and, say, ask a question, the first thing that Knowledge Buddy does is it goes out to that vector database and it pulls back all of the chunks of content that could possibly be related to the answer to that question. And now is when gen AI gets involved, but before Knowledge Buddy actually goes and calls gen AI, it constructs a very specialized prompt, a very specialized request to gen AI. And it says in that prompt, "You're a knowledge buddy, I count on you to answer questions. I'm gonna tell you the question I want you to answer. I'm also going to give you all of the content I want you to use when you answer that question. And if you cannot find the answer in that content, I want you to say, 'I don't know.' If you cannot figure out what the question means based on the content that I've provided you, I want you to say, 'I don't know.'" And that I don't know becomes really important because I'm effectively telling the model, "Don't hallucinate." So, we take that prompt and we take the chunks of content and we send them off to gen AI. In this case we're using OpenAI models from Azure. And the response then comes back, and Knowledge Buddy returns that response in the form of an answer. An answer, by the way, that is directly tied to the content in your repository.
So, when we go back and look at sort of the three challenges or three needs when using generative AI at the enterprise level, does Knowledge Buddy with this RAG approach give you traceability? Absolutely. We know what chunks of content were used to answer the question, and we know the got documents, we can even give you the URLs to the documents in your content repository that we used to answer that question. Do we eliminate hallucination? Absolutely, we tell OpenAI to say, "I don't know," if you can't find the answer in the content that we give you. And is your content and knowledge up to date? Yep, because if you have new content or you update a document, all you need to do is update the Vector database. You don't need to retrain a model, you don't need to fine tune. In fact, because we have built Knowledge Buddy to operate at the kind of enterprise level that our clients run at, we've given you out of the box all of the workflows for adding new content, managing this vector database, setting the size of your chunks, tracing all of the work that happens, auditing every question that get asked and the answers that returned.
So, with Knowledge Buddy, you've got an enterprise-ready approach to turning all of your mountains of enterprise content into immediately useful answers for your employees and for your clients. If you're looking for a way to start applying generative AI to deliver better employee and customer experiences and more efficiency today, and you wanna do it in a safe, responsible way, check out Knowledge Buddy and the underlying RAG architecture that it uses.
Tags
Related Resources
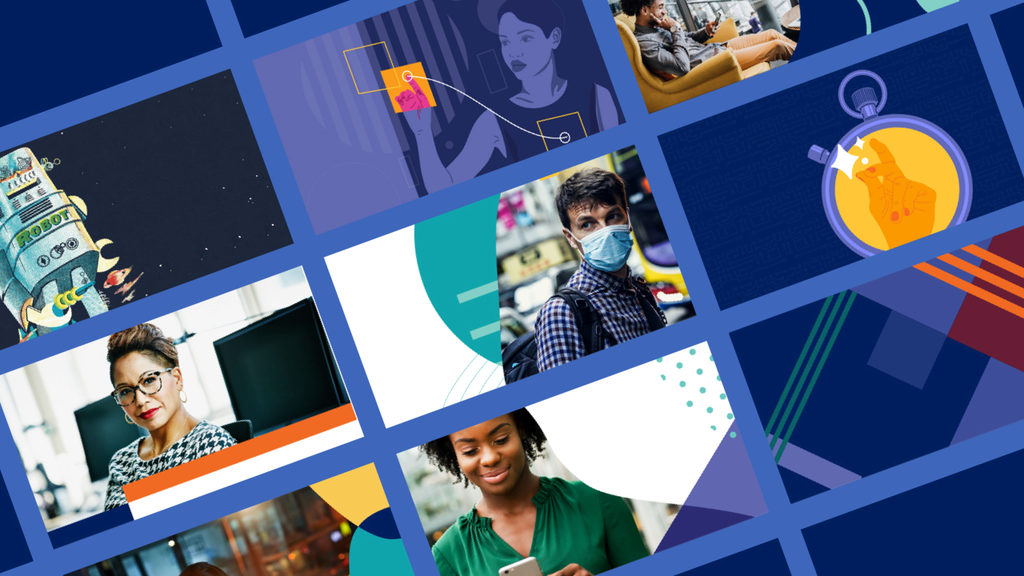
Recommended research & insights
See what’s new, what’s next, and what’s trending right now.
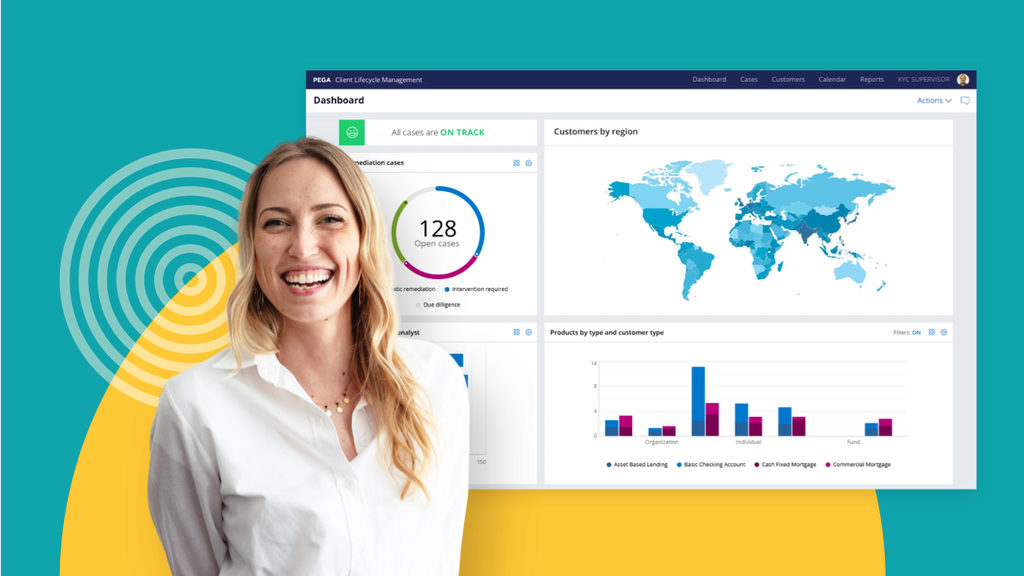
Why Pega?
Uniquely powerful software isn’t the only thing that sets us apart.