Webinar
Always-On Marketing
The pressure on marketers just keeps growing. The average campaign response rate hovers around 1 percent – at best. Customers are being bombarded with irrelevant or duplicate messages – effectively training them to tune everything out. When that happens, marketers lose the ability to reach them. But how did we get here?
In this webinar, we’ll compare traditional marketing tactics like campaigns, segmentation, targeting, and optimization with their more progressive, real-time counterparts. We’ll explore the history to uncover how a few simple changes in your approach can be a game changer for building long-term customer relationships including:
- Why do traditional campaigns fail to generate more response?
- How does an always-on approach differ from campaigns?
- How does real-time personalization work for email or mobile?
- How does a one-to-one approach drive better ROI?
Transcript:
Welcome everybody. Thank you for tuning in. Today we're going to talk about how innovative companies are pivoting to an always-on marketing approach across their outbound programs, getting a five to six times higher response rate, building tremendous customer equity as a result. The goal today is to give you a sense of where the market is headed and for you to walk away feeling empowered to engage customers in a more dynamic one-to-one way. So my name is Priyanka Raj and I am a product marketing manager here at Pega. And with me is Matt Nolan, who is the senior director of decision sciences here at Pega. Together, Matt and I will highlight the big challenges companies are facing with traditional marketing and explain why they're struggling. We'll break down what always-on marketing is, why it works, and what kind of outcomes companies can achieve if they do it right. Then we'll show you some examples of companies already out there doing this today. I'm excited, so let's jump right into it. Just to set the stage for today's conversation. As marketers in the traditional model, we've always relied on segmentation to drive our campaign targeting strategy. So imagine this is our customer base and when we run a campaign, when we run a campaign, we pick a product, filter out the list of people in our target demographic and geography with the means to make the purchase or who maybe own certain products and who have shown an interest in what we're selling. Essentially, the goal is to always find the best possible list of people ready to buy. So we take that list and then we drill into it and target those segments across different channels. And then we make outreaches to everyone in the campaign staggered over a period of time, and when it's all said and done, we'd end up with maybe one to 2% of those targets that actually responded and made a purchase. We'd done a ton of work and spent a lot of budget and maybe that was enough to hit some of the short term revenue goals. But over the long term, that approach completely devastates our customer relationships because 99% of the time they'd ignore everything we had to say. And we're essentially treating these people like they're nameless and faceless and training them to ignore us and that what we have to say has no value. So those messages don't connect for a lot of different reasons. Some weren't relevant. Maybe we had a mismatch in context, or maybe that customer would've responded if we reached out at a time when they were ready. But traditional marketing doesn't work that way. Campaigns are built completely around our timing as a business and what we want to sell, not what individuals actually need in that moment, which isn't usually a sales offer, right? So that shows a big lack of empathy from businesses. Customers feel that. It's why marketing has such a bad reputation. So if you step back and look at the big picture, the average consumer gets bombarded with more than 6,000 advertisements a day and they have to protect themselves against all of that noise. I know we all have an email account that we stashed full of junk, at least I do, and we install ad blockers on our computers. We did a study at Pega and 68% of customers actually think that businesses aren't capable of empathy or understanding what a customer actually needs, much less acting on it, and they actually compared marketers to sociopaths. So that perception obviously has huge implications on loyalty and it's pervasive. So I don't know any company that is exempt from this. So Matt, let's start there. You've been in big time marketing and customer engagement programs for a long time and you've been consulting with clients on these topics every single day. What kinds of issues does this cause with longer term engagement?
Well, first, lemme say thank you for having me, Priyanka. I'm really happy to be here. And I think what you're saying about empathy is very, very true. So it's really hard for organizations as large as companies kind of are today, if you think about it, a lot of org, they have millions of customers and they do billions upon billions of digital interactions every single year with those customers. So it's hard for them to really know somebody, one-to-one at that scale. So they end up talking a lot more than they actually listen. And those communications, like you said, they end up being very, a lot of times not very relevant. So what you do is you end up putting the ceiling on your relationship with a customer. So if somebody is disengaged, if they don't hear you, your value as a marketer drops to like zero. So, and your brand end up ends up getting commoditized and you end up being very easy to replace and developing any kind of deeper or more loyal customer relationship it becomes kind of a non-starter. Like you agree with that? That's what I think, Priyanka, you agree with that?
I do actually. And just to give people some perspective, you're out there talking to marketers and customer experience practitioners all day every day. How are the successful, innovative companies doing things differently today?
Oh, well that's probably the heart of what we want to talk about today, right? So I think I see great marketers moving from the left hand side of the equation, which you're seeing on the screen where they were running campaigns that kind of picked a product first and then used segments to kind of target specific groups of people in these big batches. They're moving from the left to the right hand side where those same marketers end up in this always-on type of mode where every time they get a new piece of data, they're looking at the individual like, I didn't mean like at Matt, at Angus, at Miranda. And they're using real-time analytics to figure out what do they need right now? I mean, do they need us to, does she need us to reach out and try to retain her? Do we have to try to help her fix a service issue? Like how can we help her, you know, and then we reach out and they reach out and they solve this problem right there exactly when she needs it. And I'll give you an example. Like imagine that you get a chance to interact with a customer. And before they were nameless and faceless and part of a segment, I totally agree with what you said there, but now you're gonna up treating them like Miranda, like a real person, right? With very, very unique needs. So when the time comes to engage with her, you look across all the different conversations you could have with her, right? All those conversations are always-on and there might be hundreds of 'em. They could be sales offers and they could be retention types of packages and service nudges, nurture messages, that sort of thing. And you score all of those conversations for propensity with predicted models or machine learning models like adaptive models, so you know exactly what that person's most likely to respond to and what they're gonna care about right there in the moment. And that conversation, the one with the highest propensity, becomes the next best conversation or the next best action. You hear people call it different things, but next best conversation, next best action are pretty common. And that conversation is what you present to them in channel, like on the website, in email, or wherever you interact with 'em, it could be anywhere. And because it's propensity driven, it drives your engagement and your conversion rates through the roof because it's a lot more relevant than what you would've get using a traditional kind of segment based approach. So think about it this way, instead of just kind of pushing an offer at somebody, you're listening, you're adapting, and you're having a real conversation to interacting kind of in the moment. And that's a very, very different thing, at least from my perspective.
That makes sense, Matt. A lot of people watching today are very focused on outbound marketing, and we covered how it works in an inbound channel, like web or call centers. But talk to us about how it works for an outbound channel, like email or direct mail, channels that are normally initiated by the marketer rather than the customer.
Okay, so great question, and that's a good perspective. So here's how that kind of works. So organizations that are running and always-on marketing program and outbound program, so what they're gonna do is they're gonna monitor each customer's context, right? As data comes in from them. And they're gonna basically, usually they analyze the data sources you see over on the left, as they come in kind of in real time. And what they're doing is they're looking for signals about what Miranda or Matt or Joe or whoever, what they're really interested in as an individual. And that kind of behavioral data, like what you're doing in interacting with on digital properties, especially, that's the fuel that you use for a great always-on program. So let's say that Miranda like browses on the website, and we take that browsing behavior, you run it through all your models right there in real time. And our home equity propensity if you were a bank, would, you know, jumps to the top of the list. So that becomes your next best conversation. But you don't wait until you get a campaign a week down the road or two weeks down the road to send that, right? You're either gonna trigger it right there in the moment by pushing it out to the channel. There is seconds after that happens or minutes or you're gonna wait to do it in your next outbound run, which probably happens later that day. So what you do is you look at all the different channels available and you trigger outreach on the channel that Miranda actually, you know, that one that she prefers, that she's gonna respond in like in this particular case, SMS, right? Like a lot of SMS messages in the real world, those go out in real time. Same thing with channels like MobilePush, right? Because of the way they're designed, because of the way that they're used. But this channel could have just as easily been an email, could have been a direct mail piece or whatever channel she was gonna likely respond in. Now every time Miranda or anyone else interacts, we're gonna go through the same process again and again, every time we get new data about her. And we're gonna use that contextual data, that context, what she's going through to power all our models, and we're gonna pick that next conversation and then deliver it in the next run and the next and her next best conversation is gonna constantly pivot like throughout that, like if you're doing like localized marketing, good example. And Miranda enters a location where we've got an event. And she tends to respond to that kind of thing. So we just reach out and we let her know. And we show her that here's something you might be interested in, no strings attached. Miranda, we're not trying to sell you anything. There's just an event might be interesting to you. And there's a million use cases for that kind of thing. You could reach out with like a retention message or an offer or a service message, a nurture type thing. Just messages that get driven by propensity when she needs them. Those kinds of touches are a huge deal to people and it's because they connect to their lives and what's going on and what they care about. So they're super, super relevant and a lot of people worry about whether they'd have enough insight about their customers to make that kind of thing work. And I hear it a lot when I talk to clients. They're like, Matt, like a lot of our customers don't interact at all, right? Like we never have enough data to manage or engage that way. You know, I explain to 'em like, you're thinking about this wrong. The goal is to constantly kind of test messages, let the AI let your decisioning engine kind of figure out what people need. So when the customer isn't interacting, that's just a context clue. You can, and you can take that and you can pivot and you can try something different and learn about them all at the same time. Like a lot of different organizations will put a nurture strategy in place that gets kicked off automatically if the customer hasn't interacted in a while. Say it's been a week, automatically you're gonna send them something, you're gonna pick the next best conversation, you reach out to them in their preferred channel, try to spark some engagement, and they do that over and over and just focus on being as relevant as they can and trying to drive some engagement and saying things that people care about. And when you find what works, you just kinda lean into it. And that's basically how always-on works. When something relevant comes up, you trigger it right then, and if it doesn't work, you pivot to the next thing and the next thing. Does that kinda make sense, Priyanka?
Yeah, absolutely. I think this is fantastic. And if you don't mind me playing that back to you, what I'm hearing is that you literally have a library of hundreds of different messages active and available at any time with models for each one, and you're constantly checking a customer's propensity to respond to them and then automatically triggering messages as they become relevant. But how does that library actually work? Is that something that you build all at once or over time?
Ooh, that's a good question. And that's actually a really common one that you get from a lot of clients too. So any company that's kind of using an always-on approach will build out a really robust customer like an action library, a conversation library like you said. So the goal is you wanna have a conversation, you wanna be able to have a conversation with a customer in almost any kind of context or any kind of situation. So for some organizations there that might be like dozens of conversations. For others it's like hundreds. And then for some it's thousands, like literally thousands of things that are always-on kinda waiting to be triggered when you need them. And the idea is that all those messages are part of like you're always-on library, right? And they're all queued up and they're ready to fire when you need them. Now generally, most organizations start with the kinds of conversations you see in the first two columns. That's why I put the thing around them. Acquisition and cross sell because those are the kinds of sales offers, the sales messages that dominate most marketing campaigns today, people are already used to that, so that's usually where they start. Unfortunately, those are also the kinds of messages that fatigue consumers, customers really, really quickly. Which is why a lot of organizations end up with a big percentage of their customer base that's unengaged, or that ops out or that routes their messages. Junk mail accounts like you were talking about a little while ago, Priyanka, I was nodding as you were saying that. And that's one of the biggest problems in marketing. And if you're a marketer, you've been there, like we all have. Now as an organization evolves a little bit and really starts to embrace more of a customer relevance and an empathy based kind of approach that what they do is they infuse those day-to-day conversations that we're having, the acquisition and cross sell stuff with the kinds of messages you see on the right. And they're things like service nudges and retention and loyalty messages and nurture streams. And like in this era of Covid, you see a lot of like resilience focused outreach that tries to get people over the hump financially and back on track, and keep them paying their bills if you will. So those are the kinds of messages that help build deeper, kinda longer term relationships that keep you really relevant. And companies usually build those out one strategy at a time. So they start with sales offers, like I said, maybe with retention offers and markets like communications, you see that a lot. But then they build out service and they build out nurture one piece at a time. It all depends on kind of what's important in your business and basically what your priorities are, if that makes sense.
Yeah, that makes a ton of sense. And could you talk a little bit more about how the analytics work? That part is very interesting. Most of the time you see model scoring happening overnight, but it feels like here it's being done in real time.
So this is, and this is like kind of part and parcel to my heart. This is where I came from and I'm an analytics guy at heart and I think this is where all the real relevance comes in. And I talk about relevance a lot because it's such a critical factor, especially right now with all the digital crap out there. It's really, really hard, you have to get people focused on what you're saying, being relevant is the most important thing. So understanding each individual, especially like at the kind of scale we're talking about that requires realtime propensity modeling, if you will. And that sounds a little scary and that feels a little bit hard, but it's not rocket science so just stay with me. So basically you need to be working in a platform or a software that can do time scoring. So if you're thinking about it, just know that that's gonna become a priority as folks move forward. Like at Pega, we do that with our customer decision hub. But there are others out there that can do that same types of thing, lots of them. So what you have to have is you have to have at least one model for every action or conversation you wanna have with a customer. So in that model tells you how likely somebody is to accept that thing you're gonna put in front of them, that offer or that message. And you can use that likelihood to look to compare all the different options and pick the one with the highest propensity. So organizations use their own predictive models, like the stuff that gets built by your data scientists or by your analytics teams, by statisticians. And you're called a lot of different things in a lot of different places. But usually what what you see now is that they use machine learning models that are created by the software they're using. Like at Pega we call those adaptive models, or machine learning models. And for us, what we do is we just generate 'em automatically when you create a new conversation. So you're gonna create a new conversation, it automatically generates like analytics go with it, and then that gets scores in real time that gets scored in real time. And that model actually updates itself so people don't have to maintain it. And the marketer doesn't have to think about it. I think that's one of the biggest piece, one of the biggest pieces here, and that's what a lot of marketers want, is they wanna know their message that they're triggering is relevant. But beyond that, they're usually okay with letting the decision and you kind of decide what to send once you prove that it actually works. But it's one, basically, think about it this way, one action, you need at least one model. And a lot of times those are machine learned, especially to get to the kind of scale we're talking about with this.
Yeah, that makes a ton of sense. And then with all of the analytics to run and so many, could you talk to us a little bit about how to keep scoring manageable so we aren't running hundreds of models at once, and how do you manage things like eligibility rules where only certain customers are allowed to get certain offers or products.
That's important too and I think because if you think about it, what can happen is a lot of people think this. If you have a thousand different actions out there and you have to run models for each one of those, every time you interact with somebody, it actually doesn't work quite that way. So, and it's important to explain. So in an always-on type of environment, organizations use rules what you hear commonly referred to as engagement policies. They use those policies to control who's eligible for certain kinds of offers, certain kinds of actions, when those people can see them and whether they're actually suitable or in a certain context. Like, and doing this kind of stuff was really hard when every channel ran in a silo or when every channel kind of controlled itself. But when you use a more centralized model where all your decisions get made in one place, like through a decision engine, it actually becomes pretty simple to manage. And what you do is you define rules like, okay, only people in these zip codes can get this specific action. Or only show this treatment if the customer already owns this or product A or product B or product C, right? Or something like a never show offers to a customer who's in bereavement status, which should just be a very simple, straightforward thing that everybody does. So every offer, every action has a set of rules and sometimes like whole categories of messages like retention have their own set of rules, right? The trick I think is not to lean on those rules too hard. So if you really want, what you really want is you want propensity to guide what you show somebody. To keep it relevant. So if you overdo it with rules and you take out all the different options, then you know you can keep the customer from seeing the most relevant stuff and that's not what you wanna do. So lots of engagement policies kind of keep you from having to process all those models all the time. Does that make sense, Priyanka, did that answer that question?
It absolutely does. I love that explanation. And just to break it down for everyone and to summarize what we just talked about, number one, a company starts introducing more empathetic actions and they've got at least one model for every conversion. And number two, they're using that propensity to figure out which conversation to trigger for a specific customer at a specific moment. And then number three, they're using engagement policies to control who's eligible, who's not, who's suitable, et cetera. And number four, not too many rules because you start diluting propensity, right. And so that makes sense in theory, but I'd love to hear more about what's actually happening behind the scenes when you trigger a message like that.
Okay, yeah and this is, I think this is good to show too, and I can walk through that. So let's say I'm interacting with Amanda, right? And Amanda could be any particular customer, but in this case it's Amanda and she has very specific things that she's interested in. So what we wanna do with any programs, we wanna find something to talk to her about that's relevant for her. So let's say we have 10 things that we could, 10 conversations that we could have with her. And in reality there's probably a lot more options. You usually have at least 50, but it could be thousands. But I've got limited space on my screen so I can't show you all that. So you've got a few sales offers, you've got a few service messages, a few hardship messages, retention offers, some nurture content, and let's say a new piece of data, any new piece of data could come in, doesn't really matter what it's, but when that happens, we're gonna rescore Amanda's portfolio, her whole profile. So first we process all those actions through rules, like I talked about those engagement policies. And some of those actions are gonna drop off 'cause the customer just isn't eligible for them or because that action wasn't suitable in the moment, right? So they get removed from that decision right up front. Priyanka, when you were asking about how you manage the amount of analytics, this is how. You're taking some options out, so you don't have to waste time, waste computing power gathering the data form or powering the models, right. Then what you're gonna do is you're gonna take those actions that are left, take all the data we know about Amanda, we're gonna use our propensity models to figure out how likely she is to respond if we presented that action to her right now. And that's the P column, the propensity column. Every action gets a propensity score and you can see which ones are really relevant, which right now which ones aren't, like it pops right out to you, like the oh 0.1% for a loan offer versus 21% for a new mobile app, 63%, 64% for other things. That's really, really common. Then what we're gonna do is we're gonna do that same kind of thing where we're gonna make a calculation, but instead of propensity, which is all about like kind of what the customer needs, we're gonna score each action for value. Which is what the business is looking for, financial value. So we're basically, we're answering the question like, if this person accepted this action, this offer right now, what would that be worth to us? If Amanda did that, what's it worth to us? And that's important because sometimes like things like a mortgage or a new iPhone or a new car or a certain policy that are worth a lot more to us than other ones are. If the customer accepts 'em, so we have to account for that when we make a decision about what to show somebody. But third, what we're gonna do is we're gonna lever up actions that really need it in certain contexts. Like for example, we might lever up things like hardship actions when that person's having a hard time paying their bills. This was really, really big during Covid, it's really, really big right now. And in this case on the screen you lever up the action of a hundred percent. It becomes twice as likely to be selected. So certain companies will do that for retention or for service or for a new product launch messages or just kind of mix and match that a little bit. It depends on what they need and what their immediate or long-term goals are for that. Now lastly, the decision engine's gonna multiply those together. And this is what happens in analytics all the time, is you're going propensity times value times lever, we call it P times V times L most of the time, and they're gonna calculate a new next best action score for each action for Amanda, right? That action is what you present to the customer and you do that every single time you get that new piece of data. With contact with those engagement policies in the background, kind of controlling how often you actually talk to customers so you don't actually overexpose them. Those are actually called contact policies. They kind of keep you from talking to somebody five times a day, you know. And that's the gist of how it works. Does that make sense, Priyanka? Does that kinda get what you were looking for there?
Yeah, absolutely. I think this is excellent Matt. And between the different channels and the data and the decisions, if you look at how it all connects, it's actually pretty straightforward, but I'm kind of wondering what it looks like when it's all hooked up to the channels. Could you show us how it all works together when all the different pieces are integrated?
Absolutely. And this is what I always talk about with marketing technology people, because that's what they wanna know. It's kind of what's it look like? What's the market architecture look like? So here's a basic kind of market architecture for you. So imagine you've got your realtime channels over on the left, a lot of times those are just inbound channels, right? Your outbound channel's kind of over on the right and your decision engine's kind of in the middle ready to orchestrate all these customer conversations. So now the first thing that happens is people navigate to your inbound channels and they interact in them, right? And they look at, they do things like they look at your webpage, they look at your offers, they look at your content, they use your mobile app, they talk to agents in your call center, or maybe they don't do anything at all. That's okay too. Like they don't have to. Now those channels are gonna call into the hub in real time as somebody lands like as the, basically if somebody lands on a webpage, it's gonna call in, call into a hub, and basically it's gonna send them back in, next best action says, show this person, Amanda or Miranda, whatever it is, show them this content or talk about this thing. And then that channel's gonna present the content, gonna present the content to the customer. Now the hub stores, all those interactions, all the responses, and it regularly goes in and crunches them, specifically looking for things to talk to those people about. And when it finds valid next best actions or next best conversations, it starts building a queue of customer email messages and SMS and direct mail all kind of out there getting ready to be released. Basically it's taking the things they're interested in and they're looking for and they're converting them into outbound messages and kind of stacking 'em up and waiting for an opportunity to send them. And they could just send them in real time, right? And a lot of folks do that, especially like I said, for MobilePush channels or SMS. But many channel providers, like especially in email or direct mail, they have an SLA, right? Like your email service might do two runs a day, your mobile provider might do three or four, your direct mail provider, maybe one big one. But when it comes time, regardless of whatever the SLA is, those queues get released out to the channel. And the channel delivers that content out to the end customer. So, and then ultimately they collect those responses, they report them back to the hub. So from the first interaction in the inbound channel to the outbound delivery to the customer in any kind of responses or conversation, it's all a very tightly closed loop where every interaction's kind of informing the next one and the next and the next. And because you're using things like machine learning behind the scenes and it's constantly looking for patterns in how people interact and rebuilding itself, every response actually makes the system smarter. That's kind of the ecosystem and how it kind of all works together, Priyanka, does that kind of get to where you're going?
It absolutely does. There's a couple of things that I think would be good for the audience to know about. First, I see a lot of clients that approach outbound completely through a journey lens and they put together workflows like this one to try to engage the customer and create a dialogue. How well does that approach work in your view?
Okay, so there's the, that's a tricky question to answer. Lemme say it this way. This kind of approach to customer centricity. This is really trying to become more customer centric. That's the goal. It's a big step forward from that old campaign methodology that we're kind of batching and blasting, right? Because it's trying really, really hard to be relevant and to trigger dialogues based on what customers are doing. And that in itself is smart and it's good. The problem is is that something like this, typically this kind of flow is only outbound for the most part. So it doesn't connect conversations that are happening on your inbound channels. And that's where most of your really, of your conversations really take place. Whether it's on web or mobile or when you're talking to a rep, things like that. It's also, the problem here is that it's usually rules based instead of propensity based. So it's really, really hard to scale that or keep anything relevant. And people, honestly, people are just more complicated than that nowadays. And you labeled it, I laughed when you loaded it, but you labeled it the journey to nowhere over there. And I love that, right? Because it's very, very true. It's kind of you're pushing people down a path they don't wanna go. Is that, you know, I love that when you put that up there, lemme just say that.
Yeah, it's funny, right? And I mean this whole concept of journey orchestration is really popular right now, and marketers have a need to prioritize and uplevel certain high value journeys. It's not something they can just ignore. So tell us a little bit about what would help them be more successful.
Oh, I think that's fair, Priyanka. And I can tell you, I can tell you what I see people doing and succeeding with. So this whole concept of a journey is a little wonky because it assumes that a person can only be on one journey at a time and like, and that nothing else is actually going on while that's happening. Like, it's all happening in a vacuum. And that's crazy man. Like if you really think about the average person is on like seven or 10 or 12 different journeys, you know, everybody's trying to do 50 things at once, especially nowadays, where we're so connected all the time and a lot of those have nothing to do with a brand really. There are places where we can lean in and we can help them and we can earn some relationship, earn some relationship equity with a person, just provide, probably providing some value in something that they wanna accomplish. Like, so I like to think about a journey more along the lines like this. So say you've got Miranda in the middle, and there's 12 different things that she's trying to get done and it's everything from like paying her mortgage or going back to school, planning for retirement, trying to figure out what to do with mom at the end of the day because she's getting a little bit older. How do you help her right now, what the best marketers do with this sort of thing is every time they get a chance to interact with somebody like Miranda, they look across all of these journeys. Not just about one, but all of them. And they see where Miranda is within all of them and they use that same propensity driven approach I just showed you before to prioritize which journey to engage her about right now. And then they're picking the journey that makes the most sense for them and for the customer at the same time. And they do that over and over and over again every time you make a decision, every single time you interact with somebody when they click on a link or do anything. So instead of forcing Miranda down a path, you stay really, really agile and you use that context to decide which journey to, you know, to prioritize and make a big deal out of it right there at the moment. That makes sense?
Yeah, absolutely. I think that's really key, the propensity and the real time. And for most of the people in the audience, they probably love the vision that you're laying out here, but talk to us about who's doing this well in the real world.
Okay, so I've actually got through, I was thinking about this, I've got three examples for you of companies that are doing honestly amazing things with an always-on approach. So Commonwealth Bank of Australia, Vodafone, and NatWest, and I can give you a great use case from each one. So if you start with Commonwealth Bank, so they're the largest bank in the southern hemisphere, in Australia, tremendous market share. And like a lot of banks, they get accused like everybody of being too big, too impersonal. So they've gone completely the other direction to try to connect with customers. So they use all your standard channels, but they actually emphasize outbound calling in their engagement, which nobody's done for a while, but they do, right? Because they want that experience to be more personal and more human. So what they do is they constantly rescore customer profiles throughout the day and when their models tell 'em that someone has a need or someone has a problem, they send that next best conversation as a lead to a branch representative through their desktop system. So that rep then makes an outbound call and the reps love this, because the leads are so much better than what they could have done on their own trying to manually comb through a whole book of business, because that's so hard. Before they started doing, like as they started doing this, they actually saw like about a 10 X increase in leads and a three X increase in conversion rate over the process they were using before. So it's been really, really valuable to them. Another great example is Vodafone. So they're the second largest telecom provider in the entire world. And so what they do is, and I know about them because they use Pega in this context. So they use Pega to centralize their marketing decisions. And they take the data flowing in from their inbound channels like I showed you a little while ago and use it to power their outbound channels. So they mine that data for what they call customer intent. You folks have heard of this in the market a lot. So they use that intent to power their models and select an best action and then deliver that to the customer through whatever channel that they're actually likely to respond in. Last year, their head of decisioning actually got up at the stage at one of our events called Pega Discover and they did a keynote and they talked about how just keeping it relevant like that and using, you know, a real time context was giving them a five X increase in response, right? A three X increase in conversion by doing this. And that's not small potatoes. I mean this is one of the largest organizations in the world. So for them the revenue potential is absolutely like, it's huge. And my last example comes from NatWest and they're a large bank in the UK and they mine streaming data. Like, and I'm talking about things like purchase transactions, like web browsing. What they're doing is they're looking for predictors of a customer having a problem. Because they wanna predict, they wanna predict when something's likely to happen so they can actually avoid it. So good example might be they look to, you know, try to predict whether a customer's going to call into their contact center with a billing issue. Or maybe that their card's likely to get declined the next time they're gonna use it. So they operate in what they in always-on mode, where every time they get new data about a customer, they rescore that profile and if they find a problem and it was important enough, they'll trigger outreach to get ahead of the issue. Like they'll send a text saying, "Hey, remember you've got a 30 day grace period with your payment." Or, "Make sure to get your card approved before you travel." And that kind of thing starts out really, really subtle, but they get more and more obvious as you get closer to the actual event or the problem. And the goal that they were going for was to try to keep NPS up and keep customers out of harm's way. And that kind of high empathy engagement earned them tons of loyalty with customers. What they found is their response rates shot up by a factor of six when they, you know, over traditional kind of campaign approaches because they were talking about things that their customer needed. It was all about the customer instead of all about what they were trying to sell. And that makes such a huge difference in any kind of relationship.
Yeah, I love those three and I think what really stood out to me was the loyalty, the increase in response and conversion. Thank you so much, Matt. That was perfect. So we're at the end of our time, but before we sign off, I wanna do one more summary for all of you on what we covered today. So first we covered the challenges marketers faced using a traditional approach with a lack of relevance and a difficulty building relationships. And second, we showcased how organizations are moving to that always-on model, which activates customers, customer data in real time and uses it to engage customers one-to-one with a propensity driven approach. And third, Matt covered how those organizations are leveraging a deep library of more empathetic conversations, extending their conversations into areas like retention, nurture, preemptive service and financial resistance, and their prioritizing what they talk about and by taking that context from each customer and picking a direction that adds value for both the customer and the business. And finally, I should mention, if they're setting this up right, this doesn't require you to replace your whole marketing stack. Instead it simply leverages a brain that brings all those different channels together onto a single experience. And that's good news for everyone. So on behalf of myself and Matt, I'd like to thank you for giving us your time today. And if you're interested in learning more about what we talked about today, please take a look at this paper, Crossing the Chasm from campaigns to always-on marketing. It provides details on everything that we talked about today from the data and the channels to the P times V times L decision making and the customer success stories. So with that, have a wonderful day.
Tags
Weiteres Informationsmaterial
-
Research and Insights
Die vier falschen Narrative des Kundenerlebnisses
-
Video
Customer Engagement Summit München 2019: • Capgemini Spotlight Talk: Ermöglichen Sie ein echtes End-to-End-Kundenerlebnis
-
Präsentation
Customer Engagement Summit München 2019: Integrierte Ansätze des Kundenmanagement im Vertrieb
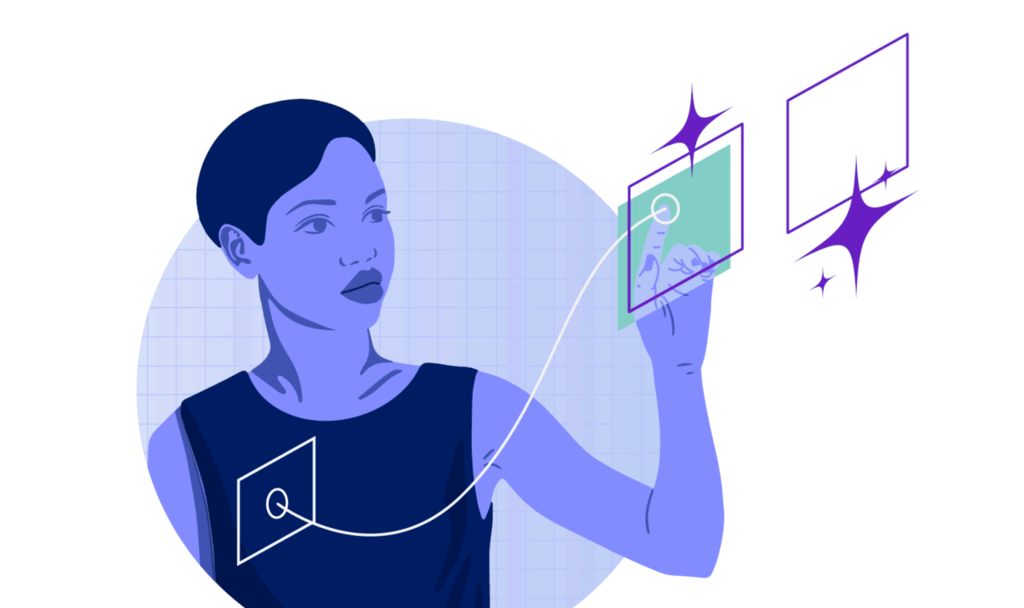
Profitieren Sie von KI-Innovationen
Erfahren Sie mehr zu Unternehmens-KI und wie sie zukünftige Geschäftsergebnisse voranbringen wird.
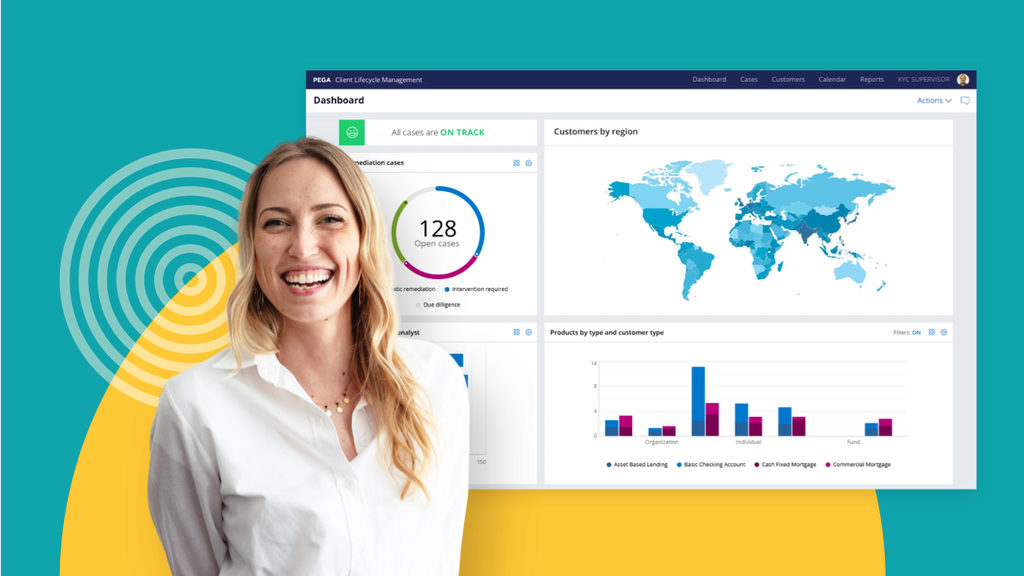
Warum Pega?
Eine außergewöhnlich leistungsstarke Software ist nicht das Einzige, was Pega auszeichnet.