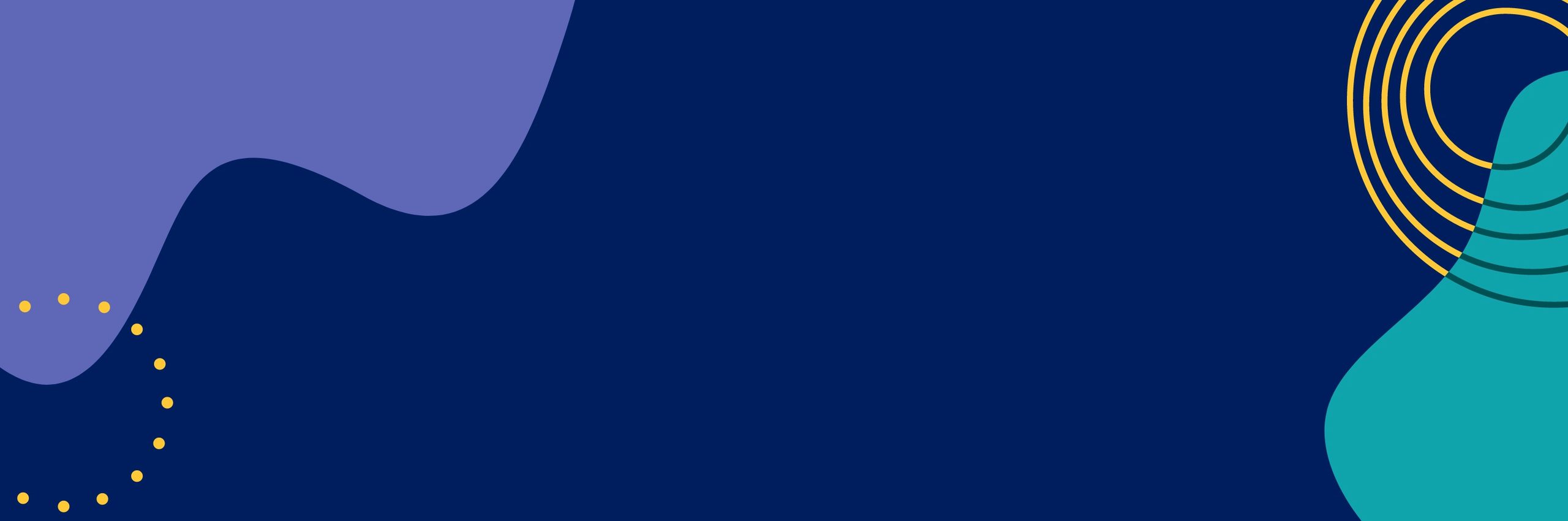
Enterprise generative AI
What is generative AI?
Generative AI is a form of artificial intelligence that generates content: from text, audio, videos, and images, to code and simulations.
As the technology evolves, it will be able to create more complex outputs like personalized marketing content, custom applications, and even software. In an enterprise context, leaders have recognized the potential of generative AI to support strategic business goals. However, knowing which opportunities to focus on and how to identify generative AI use cases can be challenging for even the most tech-savvy organization.

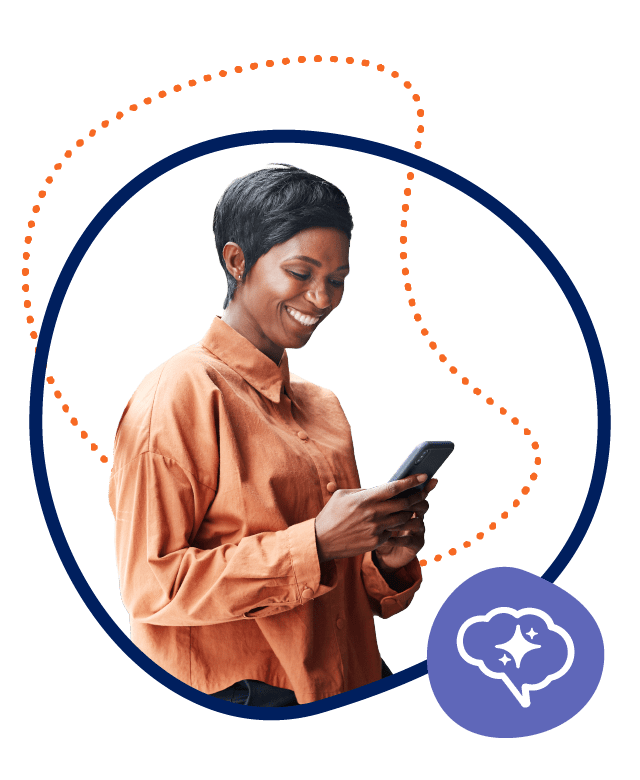
Why is generative AI important for the enterprise?
Across industries, generative AI is automating tasks, enhancing creativity, improving efficiency, and generating realistic content. Examples include medical imaging in healthcare, risk assessment in finance, personalized shopping in retail, and quality control in manufacturing – among many others.
Benefits of generative AI
- Automate repetitive tasks: AI-informed workflows cut out duplicative work so employees can focus on what matters.
- Generate personalized content: Consumers crave personalized messaging that resonates with their individual needs. In fact, a recent survey conducted by Savanta found that a significant majority (61%) of respondents leverage generative AI primarily for content creation.
- Summarize lengthy documents: Get right to the heart of the matter with quick and easy assessment of source materials.
- Keep employees informed: Offer smart pathways to information, processes, and policy to keep everyone up to speed and code-compliant.
- Improves enterprise AI: Generative AI acts as a fuel source for enterprise AI by providing the data and creative spark to train, improve, and innovate with enterprise AI systems.

How does generative AI work?
Unlike machine learning, which trains algorithms to recognize data patterns to drive predictions and decisions, Generative AI is a subset of AI that uses algorithms to create new data or content that’s similar to existing data.
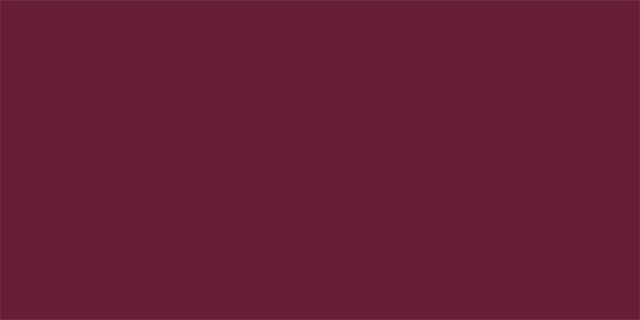
From content creation to workflow optimization, transform your business with the power of AI.

Risks associated with enterprise generative AI
Although generative AI has transformative potential, it comes with risks that organizations need to consider. While the underlying technology is similar across consumer and enterprise applications, the use cases and experiences are different. According to a McKinsey study, generative AI has the most potential across customer operations, marketing, sales, software engineering, and R&D in an enterprise context. With every organization having different requirements for workflows, business processes, and data privacy, it’s clear that consumer-grade generative AI applications will not be sufficient.
Some of the biggest risks to integrating generative AI in the enterprise include:
- Data privacy and security. Generative AI models rely on large datasets to function. It’s important that companies ensure inputs and outputs do not contain sensitive information such as private customer or company data.
- Bias and fairness. Generative AI models are only as good as the data they're trained on. If generative AI tools are introduced to biased data, their outputs can be biased too, which can result in discrimination and inequity.
- Intellectual property issues. Generative AI is often trained on copyrighted material, so it’s possible the content created could infringe upon intellectual property rights. It’s up to organizations to ensure AI-generated content does not breach any legally protected works.
- Accountability and regulation. Because generative AI is relatively new in an enterprise setting, laws and regulations have not been fully established yet. It may be difficult to determine who is liable for the output of AI or how to avoid such problems in the first place.
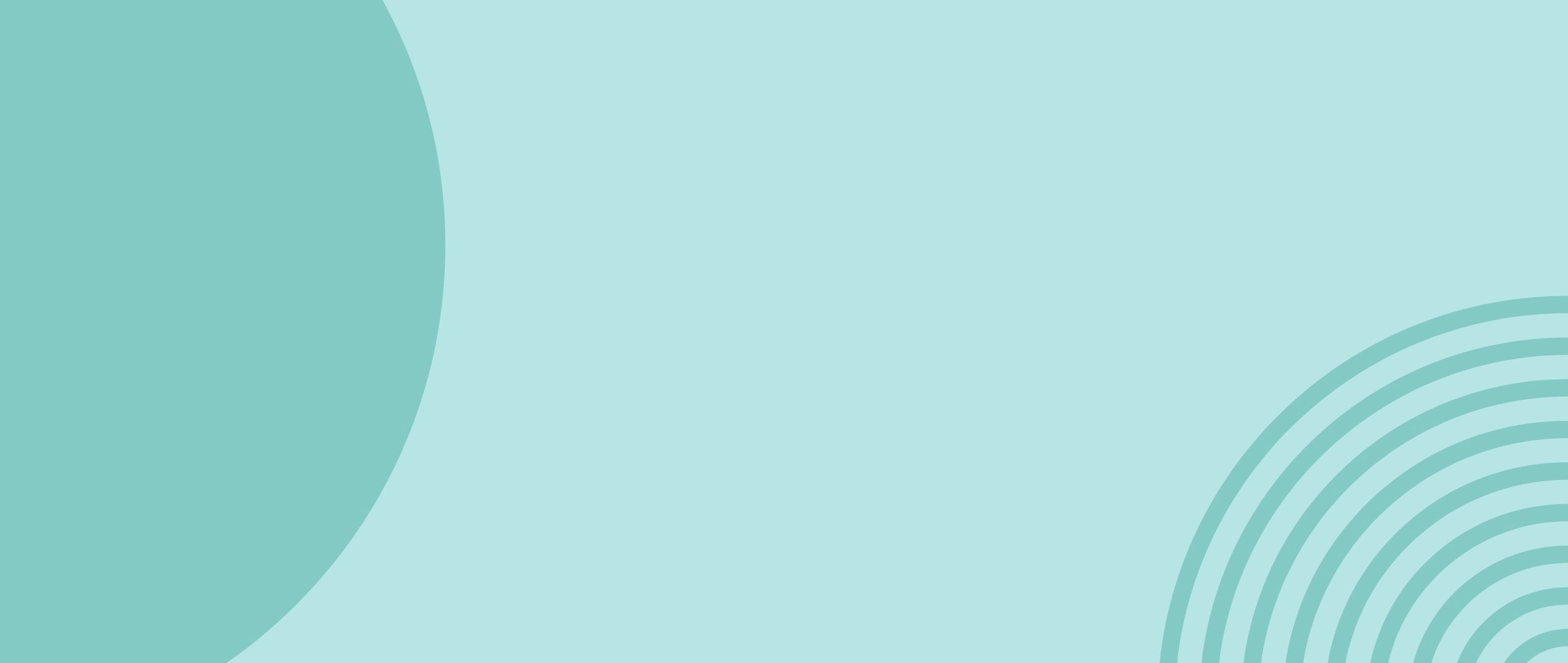
Generative AI vs. Predictive AI
Generative AI
- Generative AI creates new content based on patterns and knowledge it has learned during training
- Generative AI is more about creativity and content generation
- Generative AI doesn't require specific input data for prediction and generates content from scratch
Predictive AI
- Predictive AI uses historical data to make forecasts about future events or outcomes
- Predictive AI is more about making informed predictions and recommendations
- Predictive AI relies heavily on past data to make predictions
Enterprise generative AI use cases
Retail
Retailers face the challenge of providing personalized shopping experiences to customers in an increasingly competitive market. By using generative AI, retailers can analyze vast amounts of customer data, such as purchase history and customer interactions, leading to a hyper-personalized customer experience.
Manufacturing
Traditionally, factories fix machines when they break. Generative AI can analyze massive quantities of data to predict machine failures, enabling proactive maintenance. This reduces downtime, extends machine life, and lowers maintenance costs. Generative AI is revolutionizing manufacturing by optimizing processes through data analysis and machine learning.
Healthcare
Medical imaging interpretation is time-consuming and requires specialized expertise. Radiologists often face high workloads, leading to potential delays in diagnosis and treatment. By using generative AI trained on annotated medical images, healthcare providers can streamline image analysis, leading to quicker and more accurate diagnoses.
For further reading on generative AI
5 ways Gen AI transforms work
How GenAI & low code pay off
Why leaders are leveraging AI


What’s next for enterprise generative AI?
There’s little doubt that generative AI will continue to become further integrated into the business processes of nearly every organization. Moreover, Savanta reports that generative AI has become the predominant way of deploying artificial intelligence. From automating tasks like content creation and simplifying application development, to personalizing experiences for any kind of customer, generative AI could shape the way businesses innovate and grow.
Generative AI will likely continue to provide more value to large organizations in the form of knowledge management. McKinsey estimates the average knowledge worker spends up to one day per week searching for and analyzing documents across various systems. Retrieval-augmented generative assistants (RAGs) can create efficiency in these areas, combining the power of generative AI with search to help synthesize and summarize information quickly based on a corpus of documents. These assistants and buddies give us a glimpse toward the potential of the modern autonomous enterprise.
However, with great power comes great responsibility ¬ businesses will have to ensure they develop this technology with ethical and responsible AI practices in mind to mitigate potential risks.
Frequently Asked Questions about generative AI
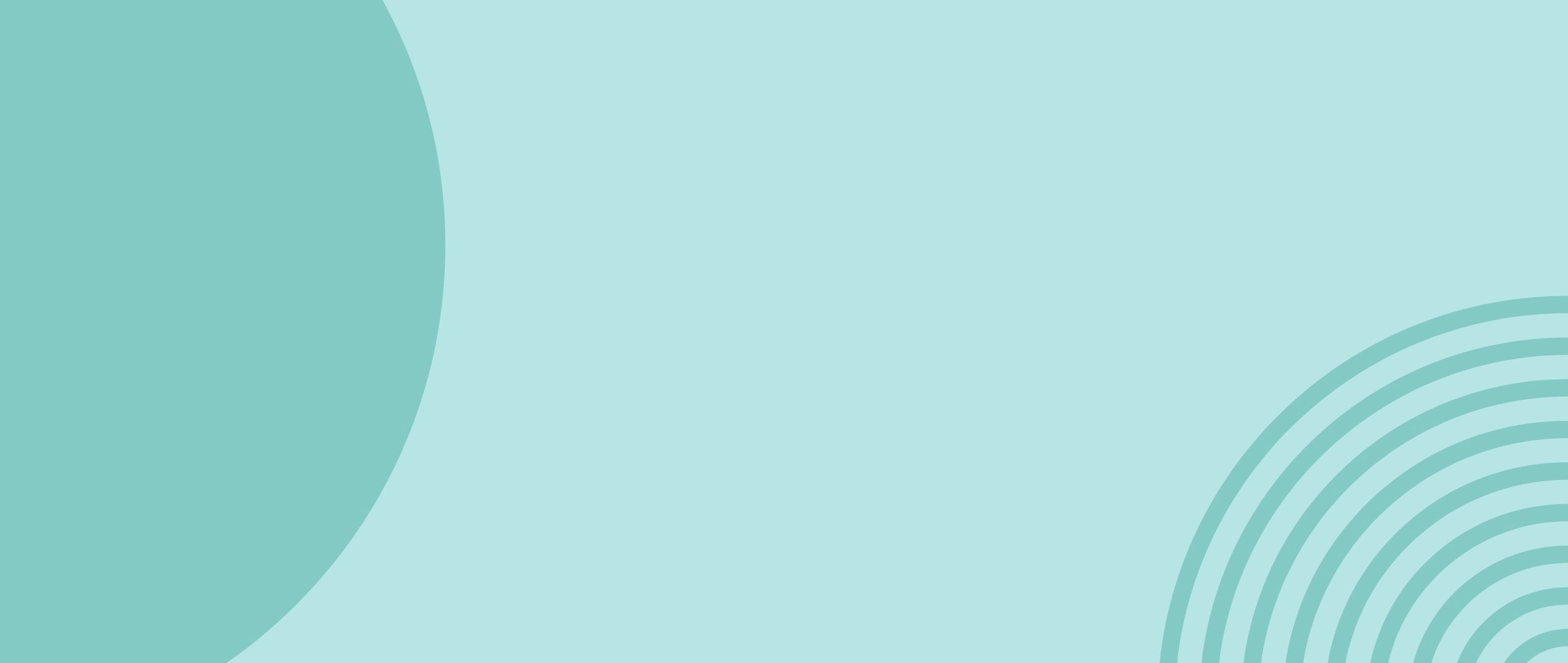