Video
PegaWorld iNspire 2023: Redefining the “A” in AI: AI and the Autonomous Enterprise
Join Peter van der Putten, Director of Pega's AI Lab and Assistant Professor of AI, as he shares his insights on the strategic role of AI in transforming businesses into autonomous enterprises. In this engaging breakout session, Peter will redefine the "A" in AI from multiple perspectives and showcase real-life examples of enterprises that use AI-powered decisioning to drive better outcomes, maximize efficiency, provide exceptional service, and increase customer relevance and value. Don't miss out on this opportunity to gain a unique perspective on the expanding role of AI in business.
Transcript:
- Today I'm going to talk about AI and how it can unlock the autonomous enterprise. Ellen spoke about the autonomous enterprise as a self-driving business, and of course, automation is important for that, but it's not enough. AI is really a must to help drive and unlock the autonomous enterprise. At least that's what I want to convince you of in this particular breakout. But it might not be the AI that you are kind of familiar with, you know, so I'm going to unpack the whole concept of AI also a little bit and hopefully give you a somewhat broader perspective on the AI that's needed to unlock the autonomous enterprise. I'm known to bring a lot of content, so this will be a bit of a whirlwind tour. So I would say, "Buckle up and on we go." Now, if you think about autonomous, let's part here from the autonomous enterprise for a brief moment. One of the key autonomous examples, of course, is the autonomous car. Yeah, and maybe just to debunk a myth straight away, and sometimes when people hear the word autonomous, they think we're not in charge. But that's not right. In a self-driving car, I would set the direction where I want to go. I want to reach a particular destination. I want to have a comfy ride or maybe want to get there as fast as possible, or an eco-friendly ride. So I set the goals of the system. And I'm basically monitoring what the self-driving car is doing. So at a high level, I'm in full control, maybe even in more control than if I would be steering the car myself. But the key idea, of course, if we can have these autonomous cars, why can't we have these autonomous enterprises as well, these self-driving enterprises. But, but let's stick with this autonomous car, a metaphor, for a brief moment. How, what kind of capabilities does the autonomous car need to have? And there will be quite similar to the capabilities that we need for an autonomous enterprise. So first off, we need to sense a lot of data. Let me get this out of the way. The car will perceive all kinds of data flowing in. And so we gave it the destination and what kind of ride we want. And then it's tracking all this information. It's not still information in databases. It's real time information that's streaming in, quite often noisy. And we need to make sense of it all. Yeah. For instance, this little blob that we see, is that, yeah. Is it just noise or is it actually a biker, yeah. That we need to make sure a cyclist that we don't bump into. And so, and things can change suddenly maybe a pedestrian steps in front of you and you need to quickly react. Or maybe a car in front of you is taking a left or a right. And the autonomous car, the AI in the car needs to observe that and needs to take a decision, what to do next and take appropriate action. And so those are some of the key capabilities that an autonomous car needs to have. And they're quite similar to what an autonomous enterprise would need. And I'm also a part-time professor at a LAD university. So I love old examples here. And this is just a quick reminder that even before the word AI existed, invented around 1956, that was the whole field of cybernetics. This, the professor that you see here is Norbert Wiener, kind of one of the main inventors of that era. And you see a little, he's holding a little self-driving autonomous car. And the key idea of cybernetics is the idea of feedback loops. Yeah, so a cybernetics system, like a self driving car, like an autopilot in a plane, like an autonomous business, constantly needs to monitor the environment, has certain goals. So it takes actions, decisions, and actions to further those goals. And that it needs to learn and it needs to take another action, observe whether the outcome was right. Yeah, so and in that sense, you know, maybe I should rephrase my lab to the, not from the AI lab to the cybernetics lab, because I think that idea of a feedback loop, that's an incredibly powerful, powerful idea. But let's get a little bit more into other examples as well. This is a great old example from the 1950s. This is CYSP, and CYSP was a robot, and this robot was built by an artist. Yeah, it's not a useful robot. It was an artwork. And CYSP could actually sense the environment. So it liked light, but not too much light. It liked sound, but not too loud. It liked warm colors like red and not blue colors. And it could also move. Yeah? So it would sense the environment and then maybe move a bit in the direction of the things that it liked or backed away. If it was carried by it. And by putting it into different environments, you can get interesting behaviors by this robot. And you can imagine if I put it in a gallery, it'll start to behave a little bit like the other people that walk into the gallery. Yeah, but if I put it in an environment with ballet dancers, the robot will start to dance. Yeah, so a little bit of a frivolous example, but again, this proves, this is the 1950s, you don't need advanced technology per se, huh? To build a cybernetic system where we sense the site and act. Okay, so this leads into talking a little bit more about the autonomous enterprise. What is the autonomous enterprise really? And so the autonomous enterprise, it applies both AI and automation to further its goals. Yeah, but a key word in this definition here is that it talks about a self-driving business. Yeah, so there needs to be an element where we, you in the room, you set the goals of what you want to achieve and the system will sense the side and act to further and optimize those goals. Yeah, that's the idea of a cybernetic self-driving system. That's the idea of an autonomous enterprise. And for that automation is not enough. Yeah? So maybe normally your current automated systems, you say, "Ah, let's plug in a little bit of smart. That's enough. It's nice to have." But in an autonomous car, you can't have an autonomous car without AI, without that intelligence. Rightfully so, in an autonomous enterprise, you really, really need that AI. AI is a must, but what type of AI are we talking about here? Yeah, of course, we all know AI is artificial intelligence, but it could mean so much more. Yeah, for example, can we make sure that we do something with that intelligence? Can we make it actionable intelligence by putting it into processes, by putting it into interactions, making sure that it has an impact. Can it be augmented intelligence working alongside a human, not replacing it? Can it be accepted intelligence? Can it be always on intelligence AI that is just everywhere and ultimately can it become autonomous intelligence? Yeah, so it's a complex multifaceted concept AI and these are different ways to look at AI. Yeah, so accepted intelligence was in there, and that's the key point around how can we make sure that AI is worthy of our trust? Yeah, Rob will be talking about it tomorrow as well, but how can we make sure that the AI is not biased, that it's transparent, that it's serving the purposes of all the stakeholders. But from an autonomous intelligence point of view, we get back to this feedback loop. Yeah, so again, you know, like in my autonomous car, I tell it where it needs to go and where it needs to be a comfy ride or a fast ride, but there's also constraints, all kinds of rules of the road and traffic regulations. And then it constantly, the car needs to make decisions, you know, do I need to push the gas or brake or steer and constantly interact and observe what is the outcomes of taking those actions? That's how we need brain and muscle. We need AI and automation to make that happen. And if I unpack that even more, and then you get into this sense, decide and act loop. Yeah, so sensing the environment, making smart decisions, what to do, taking those actions. And then the key point, you can only be a self optimizing business or a self optimizing system if you learn from that. And so take that feedback into account and actually actually learn from it. Now, and if that sounds a little bit like a grant, a vision for the future, well, it might be a north star vision, but it is something where you can get step by step similar how you think about self-driving cars. Yeah, there's a bit of a transition over those different stages. We also did a ran a survey where we asked respondents, "Where do you see yourself now?" Yeah, so you can see that a lot of the respondents here, they say, "Oh, we're either in the simple automation stage or maybe in the intelligent automation stage." But when we ask them, "Did you already start projects to move more towards the autonomous enterprise," around three quarters of respondents said, "Yes, you know, we're already moving in that direction," and the vast majority, 98% sees them to be at that stage on let's say a 10 year horizon. So everyone is thinking that we need to move there. Yeah? And if I look at a little bit of an evolution, again, you can think of, oh, you know, we started with all kinds of unmanaged tasks. Yeah. That's a mess. Let's start with automating that a little bit. Yeah, but at some point you run out of runway, you've reached all your automation benefits. So then you need to think about can we become smarter? Can we put more intelligence into those processes? Can we put more intelligence in those interactions? Yeah? And then ultimately, basically you're building a Tesla. Yeah, you're turning your enterprise into a Tesla, but who's gonna drive the Tesla? Right, so, and that's also where you get more into the autonomous, the idea of the autonomous enterprise. Yeah, so Citi, in their keynote this morning, they also alluded to we're actually building a Tesla for marketing in their example. But how are you going to drive that Tesla? Yeah, and that's where some of the additional capabilities actually come into play. Okay. So that was a quick intro of the general concept. Let me try to unpack that even more and I'll do two things. Yeah, first I'll talk a little bit more about what are some of those autonomous use cases, in engagement, in service, in operations. Yeah, so a little bit more from a use case point of view and yeah. Given the limited time, you know, I just have some hints, some examples, but as many more ways how you can build more autonomy into those processes. That's step one. Step two, I'm going to talk about the AI itself. What's the AI that underpins this and that actually makes that work. Yeah, so, and I use AI here in a very kind of loose, very looser, much more than if you would browse the internet nowadays, you would think that, oh, AI is super duper machine learning, but AI is so much more. Yeah, so I think the example, the cybernetics example that I showed, that kind of proves that point as well. Yeah, so use cases, capabilities, use cases first. Now when we think about becoming an autonomous enterprise, what can we expect? We can become smarter, yeah. In autonomous customer engagement and making sure that when we interact with customers, or actually better put from a customer perspective, when customers desire to interact with us. Yeah, that we're real time and contextual about the offers that we make to them. And that we learn, you know, which messages resonate best with these customers. But of course new AI capabilities like generative AI can also feed into that. Autonomous service. How can we not just, well of course we can provide intelligent guidance to customer service agents. Yeah, that's a great idea. Makes our life a lot better. Makes the life of customers a lot better. But if we have that intelligence, why don't we use that earlier in the process? Yeah, and the third one is autonomous operations. So how can we, and maybe we reach certain benefits, you have reached certain benefits already with automating your core customer operation processes, but then you need to take it to the next level. You need to insert that intelligence to optimize not just the efficiency, but specifically also the effectiveness, the particular business outcomes that you're trying to reach. And so I'll give some examples of those three areas and then, well, before I do that, of course, and you can see here what are the major solutions that we have for those areas. And so for one-to-one customer engagement is Customer Decision Hub. And we have a lot of smarts in terms of gen AI, self learning models that help drive relevant conversation with customers across all channels. In customer service, it's better customer service, but also specific AI capabilities like voice AI or NOP or email bots. And then in autonomous operations, things like process AI actually help drive the efficiency and the effectiveness of those processes. Yeah, but I'll give you some examples. Let's start with one-to-one customer engagement. Now here you can see kind of a similar evolution map that I paint where, you know, in one-to-one customer engagement and traditional marketing there you start with maybe automating some of the processes, but then you want to make them smarter, making sure that you actually build the Tesla and that you have a self-optimizing system that figures out which messages resonate. But then how do you drive the Tesla? And that's where autonomous, the idea of autonomous actually comes into play. And so maybe at the first level, yeah, you have all these next perceptions you can show and you use data to figure out who is going to be likely responding to what type of next-best-action. Then you track the outcome in the cybernetic feedback loop and you learn, yeah? So you already have your automated system that can automatically do that targeting, but then the next question is, how do we make that more autonomous? And we have specific capabilities that can help with that. Yeah, so let's imagine you have that engine up and running. Yeah, you feed it offers the next-best-action, it's learning the who to target with what, how can I further improve this? Now one part is maybe we want to understand are there particular parts of my next action strategies that don't work well enough? Yeah, maybe we need to make sure that certain groups are not getting relevant offers that we need to loosen our rules and criteria. Maybe we need to make sure that we weighted more towards providing the next-best-actions that customers actually want through prediction. So impact analyzer, we'll basically do your doing analysis of your self-driving system for marketing and figure out different areas where you're doing well and where you can improve. Yeah, so for example, also with value finder, you see an example here where let's say we have this system up and running, but there's a consistent group of customers that are not getting relevant next-best-actions. And maybe because our rules are too strict or whatever it is, and the system will automatically find those groups and it will then say, "Hey, we don't have, we don't seem to have good next-best-actions for our Gen Z customers or our millennials." Yeah, so, and that's where generative AI can come into play. Yeah, it can actually generate new variants of offers and treatments on the fly that might better resonate with that particular segment. Yeah, or maybe there's one particular action that we want to improve. Yeah, our next best action for offering a platinum card, again, we can use generative AI to generate new offers and treatments for that particular action, okay. So that was one-to-one marketing or one-to-one customer engagement. Next topic, autonomous service. How can we make service more autonomous? And like I said in the intro, there's a particular moment of need with the customer. And then typically what we do, maybe we call up a call center and what we can do, of course we can provide intelligent guidance, technical troubleshooting, or whatever it is to the agent to make sure that this issue gets resolved. Yeah, but just to repeat, if we have that intelligence, why not use that intelligence early in the process? Yeah, why not just before the customer dials in, maybe we can use the AI to figure out what the likely issue is. Yeah, and provide an automated solution. Yeah. Maybe we can provide a self service, but what would be even better, the holy grail of autonomous service is figuring out that an issue has happened before the customer got in touch with you, or maybe even before the actual issue has happened. Yeah, so in that sense, autonomous customer service means that we can actually be way more proactive and anticipate the customer service issues and resolve them as early as possible. Now let me give you some examples. I spoke a little bit about it since the site enacted the cell driving car that gets all this data that's streaming in and trying to make sense of it all. And you see a particular com media use case here where we're listing to all kinds of network data. And we're figuring out like, did Peter experience a lot of drop calls? Yeah, or his data is not working as expected. Now we can detect those patterns and then we can for instance, notify the customer. We are aware of your issue, even before the customer got in touch with us and we're on it. We can basically notify the network people if it's not just an issue for Peter. But for many customers like me, maybe a particular seller needs to be updated or even product managers if it's occurring, if we get frequent types of issues around particular product or service. Yeah, and this is not kind of limited to one particular industry. If I think of an automotive industry example here, how you can see it's a little bit small, but down in the bottom, maybe customer comes into a dealership and we pull all the data, all the event data that's collected by these cars, we can actually pull it in or listen to that really in real time as well. And to detect particular issues that this car may be having, yeah. Or might have in the future. And yeah, recommend that maybe to change the brakes or change the oil or change the tires or whatever it is. Now and to continue on that path. And we can use NLP also to when you get loads of inbound emails to make sense of the unstructured data, the text, any email, but also link that with all the structured data you already have about the customer and the customer service history and whatnot to make decisions about, hey, can we deal with this automatically? What would be the resolution if we can't deal with it automatically, who should we route it to? Et cetera, et cetera. And then we get more into self service. This is another cool generative use case and this is a showcase application, Pega Self-study Buddy that we built. And we basically said, "Well we want to be able to give advice on a very particular domain." Yeah, if I have a customer service example, it could be self service for Citi. Yeah, but it could also be the internal KYC department within a bank that has loads of documents around regulations and can we provide a Chat GPT style experience on top of that very, very specific domain. Yeah, because if I have complaints about my credit card for bank X, I don't want to get GPT recommendations that are based on bank Y. Yeah so, and what we did in the showcase application, we did something similarly. We said, like, "We want to have this Chat GPT experience but for Pega product documentation." Yeah, so, and we gave it all the documentation we have about Pega on Pega Community and you can just ask it questions about any Pega product question you may have and it will provide an answer. And we get all kinds of things for free. You know, I was also asking questions in Georgian the other day, or in Ethiopian or, not that may occur very often, but it's a great way to unlock the knowledge that's stored in all of those documents. Okay. So that was autonomous service. Now we're more moving more into autonomous operations. Yeah. So all these complex processes and cases that you're managing your business and how can we further improve both the efficiency and the effectiveness? Yeah. So what do I mean with that? And so efficiency is doing, you know, for example, doing things faster with the same resources, being able to deal with more requests or increase the level of automated approvals, et cetera, et cetera. Whereas effectiveness, and that's about your business outcomes. Yeah, if I have a claims process, being able to deal with more claims quicker, that's maybe an efficiency benefit and effectiveness benefit is making sure that your best customers, that you help them in this difficult time, make sure that their issue gets resolved as quickly as possible, but also make sure that you keep an eye on fraud and leakage, that you don't pay out claims money that you're not supposed to be paying out. Yeah, so with the idea of process AI, we can autonomously optimize both for efficiency and effectiveness. And you can see here when we launched it at the journalist saying, "Well, life inside the self-optimizing AI automated company," right? So there needs to be an element of self-optimization here. How can we learn from all those decisions automatically? Now, lemme give you an example. I have here Anthony Leonardi, one of our colleagues came up with this great metaphor, he calls it "The Automation Sandwich." So it's a little bit like a hamburger as you can see here, and let's say it's some kind of approval process. Yeah, so it could be claims, could be any type of approval process. And maybe today, you know, you were able to kind of auto adjudicate a bunch of those cases or claims coming in at a small level with some simple business rules. So there's still a lot of work that a human needs to look into. Now how can we use something like process AI to make that more autonomous? Yeah, what we can do, we can actually have first maybe have the AI look over the shoulder of your experienced employees, your experienced caseworkers, and see how they resolve certain cases, which cases actually should be typically be approved and which should be declined as an example. And it can learn by observing the human behavior. And then more and more as you get more confident with those models, then you can start to auto adjudicate more and more of those cases. These are, we're going to approve, these, we are going to decline. And of course there will always be an element of difficult decisions, where you want to escalate and where you want to leave it to the human. But even there, AI can help, AI can help to provide all kinds of advice and assessment to the human caseworker to help resolve that particular case. Also to avoid any biases that the caseworker may have. Yeah? So AI can be biased, but humans can be biased as well. Right, so always good to work shoulder to shoulder and have a body sitting right next to you. Yeah, and that was an example around approval. But you can imagine any process has a beginning and end. Right, so, and there's all these steps in a process where we can make particular decisions. Yeah, when the work comes in, you know, is this something we can deal with automatically? Should we route it to a human? If so, which team should we routed to, which team is most likely to resolve this or best fit? What's the complexity of this particular case? Can we forecast particular SLAs maybe? Yeah. And while we're doing the work, maybe the work might be slipping, we can predict that. Yeah, and we can then intervene. Yeah. Or there might be other reasons to escalate or investigate. In the claims example, if we spot there's maybe likely leakage or fraud that let's say a first notice of loss agent cannot deal with will escalate to some type of investigations department, but contingency also predict, hey, what are the particular outcomes of this process? How can we predict the likely outcome so that you can anticipate the outcome, for instance, cases that are going to take too long that you can take remedial action, not at the end when it's too late, but early on in the process. Yeah, now those are just some examples from a generic process, but you can imagine there's many very specific decisions for specific industries that you can, where you can use this idea of self-driving, self optimizing AI. One of our customers deciding how to best load up a plane with cargo. Another customer is looking into other particular health insurance claims where we might incur fees. If we don't deal with these particular claims quick enough. And another bank insurance company that we work with is looking at claims decision, yeah? A very typical case for this type of autonomous use. Okay, so those were some of those use cases application areas. The final thing on the menu that I promised to you is to talk a little bit about these underlying capabilities. So what in the broader sense, what kind of AI capabilities you could help to actually make the autonomous enterprise a reality? Yeah, so this is the final topic now. And I can go back a little bit to this loop again, our beautiful cybernetic feedback loop where we're sensing information, we're making automated decisions. Yeah, these automated decisions that could drive a process autonomously, but could also be advised, given to a caseworker or something like that. And then we take the actions. But like I said, it's important to capture the out, you know, what is the outcome of taking that particular action and so that we can learn so that the system can self optimize. Yeah, and things like generative AI can also play into that you see "generate" there as an element because it can, well, for instance, we can use it to come up with what are the particular actions that could be useful in a particular instance. Yeah, or even in its most farfetched form of using generative AI, we could create some autopilot that also that decide itself. Like what kind of tasks do I need to do to resolve this particular case. Right? Basically we can generate anything including plans. Okay, so let me give you a little bit of a flavor here what that looks like. If I unpack it again in terms of sense, decide and act. In the sensing part, we could be listening to all kinds of data, could be speech, could be text, it could be streaming data. And then we need to make sense of it all. Yeah? So we can use predictive analytics to make all kinds of predictions or classifications. Yeah, but we can also do things like process mining. And you saw the announcement this morning by Kareem about our launch of process mining, where you need to figure out like, are there particular bottlenecks in how work is flowing through my organization? So that's the sensing part, but you know, just sensing or just insights are useless if you don't take any actions. Right, so we need to translate that into particular decisions to take actions. Yeah, so, and that's typically what we call decisioning, but where that could be a mix between predicting what is the likely success of taking particular actions. Yeah, generative AI maybe to come up with the universe of actions that are available and then indeed the depth of online learning to make sure that we self optimize that the system can learn to predict which actions are most likely going to be successful. Yeah? So not rocket science. And then ultimately taking the action of course, then you get more into our automation capabilities, case management, robotics, et cetera. Now lemme give you some examples. First we're in the sensing part. So this is a screenshot of process mining again, like you saw earlier today. And let's imagine that this is indeed our insurance claim process. And in this case you can see a big arrow, you know, going back from the bottom to the top again. So apparently a lot of work is rerouted back. We'll get an immediate estimate how many thousands of hours that's costing us. And then we can also ask like, "What is the reason, why is that happening?" And the system will tell you maybe like, "Oh, that's because you know, this particular piece of information is missing in those cases." Well maybe then we should ask that upfront. Yeah? And this way we can optimize the process. Yeah? And we can monitor any process. And these could be Pega processes, which is incredibly powerful because we have control of all the data that's flowing in there. But we can also use it to onboard new processes. We can take logs from external systems and first understand, hey, what is the process behind these logs? Yeah, people tell you, "Ah, this is our best practice process. Well give us a lock and we'll have a look what the real process is, how is happening in the real world?" And then you can already see spot opportunities to simplify that process, make it better, and then actually also put it into Pega. At our sensing example, listening to real time streaming data. And so again, if we're still in that imaginary insurance company, claims are coming in and there may be a whole bunch of small claims about Peter. Yeah, where normally the system would say, "Oh, that's just a small claim." We, you know, it doesn't really matter, but it doesn't really have the background and that's 5 minutes ago another claim for Peter came in and 10 minutes ago, yet another claim for Peter came in. Right, so it can be incredibly important in any company to listen to that streaming data and to understand those patterns. Yeah, if there's many small claims for Peter could mean two things. You know, I went to the GP with a little cough and it turned out to be whatever, covid or something nasty. Could also mean, or I was maybe hit by a car. And then you need to be on top of it to help me and making sure that you contact the providers and making sure that I get the best top quality care. Could also be that there's an evil dentist somewhere sending, you know, little claims for pulling one tooth from Peter, but a hundred in a row, yeah. Hoping that this evil dentist doesn't get caught. Yeah? You would catch that immediately with these type of systems. Now, and like I said, other data as well, unstructured data like making sense of text, like you see here, extract important entities. What is this particular text about? What is the customer trying to do so that you can combine it with the other data that you have to make automated decisions. And because now we're moving from sense into the site and there's all kinds of decisions that we could take. Yeah, so you see a bunch of them here on the screen, but ultimately anywhere where there's repeated decisions of a particular type, yeah? They are good candidates for decisioning. Especially also if it's not like a black and white type scenario where you have multiple actions you could take and they all could be valid to some degree. Yeah, so decisions in that sense and actually make the AI real. Now an example here, we're in a particular process and we can call out for a real time decision. If this is some kind of approval process, what's the chances that this should not be approved? Because maybe it's some fraudulent application. But like I said, there at the bottom, you can see all kinds of different steps in the process where you can make those automated decisions. Case complexity, routing, case resolution, investigation, escalation, outcome prediction, you name it. And this is more in the area of operations. How about when you look at our other solutions, like one-to-one customer engagement. We have similar things where you can see here, this is our customer decision up and we're kind of defining how do I decide on the next-best-action in a particular circumstance. So we're combining, first we're filtering down all the next-best-action based on rules. And then you are left with a universe of stuff that's eligible and suitable. How do I rank that? And so we need to use the AI to figure out for each of those actions, what's the likelihood for that action to have a successful outcome, a click or conversion or whatever you define to be positive, and then weight it with other factors to priority rank those next-best-actions or in milliseconds. Yeah, so what's hiding then underneath, I'm getting deeper and deeper into the machine here, doing a little bit of neurosurgery on the brain. It's a mix of self-learning adaptive models that for each action that you take that actually learn, you know, what is the like of a successful outcome. So our customers have hundreds to thousands of those adaptive self-learning models in their system. But it's not enough, right? So just like in an autonomous car, I can take particular actions. I have certain goals, I can predict the likelihood of a good outcome for that goal. But I also have rules of traffic. You know, I also have policies that I need to take into account. Like I said, we wouldn't sell a mortgage to a 16-year-old. Right, so it's the interesting interplay of the adaptive models that take care of the self-learning, self optimizing aspect. But then the so-called decision strategies that allow you to express all kinds of rules and policies that you have on top to really make it real. Some of your decisions might be, you know, driven entirely by rules. Yeah, so, and just to give you like a little bit of a tangible flavor, what that could look like. Here we are in prediction where we have access to all of our various types of predictive models. Not just Pega models, but maybe models from AWS or Google or you name it. And you see a screen here with tons of little bubbles. Each of those bubbles is an adaptive model for the different types of actions you can take. And we provide a lot of tools nowadays for your analyst to analyze the performance of those models and understand like, hey, you know, what's driving these decisions? What's driving a good outcome for a particular prediction? So we can really drill down deep. They can do it in Pega, but they can also use their favorite tools of choice. So we have a extensive Python notebooks on GitHub, for example, that your analyst data science could use to analyze these self-learning models. And then finally we get into act, you see the example here again, that Kareem also showed where we're generating a process for a loan application in this example. And so I thought it was useful to repeat that. You can see here it's creating the stages, creating the steps. We can change that of course if we don't like particular stages or steps so that we can tune this application towards our needs. Yeah, and then it will generate all the underlying things that we need as well. Like generating a data model, for example, generating personas, pulling in recommended APIs to call, you name it. And we'll have a runable prototype application that you then can change towards whatever your needs are. And because it's already living in this low-code platform. Yeah. So we went from sense, decide and act. In that sense, we're kind of completing that entire loop. So I hope that I've shown a little bit here, you know, what the autonomous enterprise is and also why AI is actually a must to drive and unlock the autonomous enterprise. Gave you some examples here where you can use those concepts almost from the point of view of, yeah, maybe you already have like a self optimizing process, but how would you make it even more autonomous? And what are some of the AI technologies? We have many more that underpin this. Yeah. And to that point, if you want to start experimenting with this, I would encourage you to become an autonomous enterprise. You start to think about it, our survey has shown that 75% of the respondents we asked, they're planning to become an autonomous enterprise in the future. And they have concrete actions that they're already taking to that point. And the other point I would like to make, you saw some sprinkling of generative AI examples in this presentation. I try to keep my powder dry because tomorrow at 2:00 PM we'll have a breakout purely on generative AI. Yeah, so if generative AI is something that interests you, check out the both demos, but also come to our breakout tomorrow at 2:00 PM. And with that, I'm at the end of my presentation, we still have over 5, yeah. A good 5 minutes or so for questions if there's any questions from the audience. Yep?
- [Audience Member] Can I
- Yeah, so the question was AI, interesting. Yeah. But how can you make sure that you use it in a responsible, trustworthy manner? I think that you are pointing to that. Yeah. That's really important. So we have transparency, for example. We can automatically generate explanations for predictions made by these models. But you can also use these, for instance, these decision strategies to really constrain whatever these machine learning models are predicting and say, well, yeah, great that this customer wants to have a mortgage for $2 million property, but you are a 16-year-old. Right? So you're not getting it, right? So it's the transparency, automated explanations, the control by combining models and rules. And thirdly, I would like to point out is also bias checking. Yeah, so we have particular capabilities in the platform to check whether there's any unwanted discrimination in your models or in your automated decisions. Yeah, so based on gender or based on age or any other group that you want to protect, you can measure and simulate, you know, is my level of bias within bounds. Yep?
- [Audience Member 2] We need several licenses
- Sorry?
- [Audience Member 2] Can you throw some light on licensing please, sir?
- Can I throw some lights on?
- [Audience Member 3] Licensing.
- [Audience Member 2] Licensing.
- [Audience Member 3] Licensing.
- Licensing. Oh, such a boring question, no. I make stuff. I'm not selling it, yeah. No, but you can see here, we put AI into, I was joking, it's a great question. We put AI into all of our products and go to markets and my job is actually make sure that we move beyond just AI and marketing and one-to-one, but use it everywhere. But that means that also we align with whatever the pricing models are for those various applications that will be different for one-to-one marketing and customer service or intelligent automation. We have different, we try to align with how those solutions are typically sold. Yeah. And then have, yeah. In some solutions it's packaged. So for example, one-to-one customer engagement, it's always there. Customer service and intelligent automation, it's more, these are options you can also license. Generative AI part, we're still, what we're going to do is we're gonna release it in preview mode in Pega 2023. But you bring your own generative AI "key" to the servers you want to use. So that will be at least what we're going to do for 2023, where we launch it in preview mode and then we will learn from interaction with our clients. What would be the best way to actually deal with that. Yeah, but we didn't want to wait with bringing it out until we sorted out those type of mundane things like licensing, yeah?
- [Audience Member 4] Performance. Is there any?
- Yeah, maybe a good, yeah, a good question. Performance, so you mean technical performance or? Yeah. So it's really important that these decisions can run at scale. I mean that's also the reason why, you know, up until two years ago, we were very focused on one area and to get it to work for their really well, which is the one-to-one customer engagement area because that's where you have hundreds or sometimes thousands of decisions per second. Very, very high volume. And so, and the idea was there, if we can deal with the performance aspects of one-to-one customer engagement, customer service and intelligent automation will pretty much be slam dunk because the volumes are way, way lower typically in those areas than in one-to-one. In one-to-one, we have customers with over a hundred million customers and indeed thousands of real time decisions per second. So very, very high volume. Okay. With that, if there's no further questions, I'd like to thank you for coming here and well happy to stay around and have a chat with anyone else who would still like to ask something and have fun at PegaWorld.
Tags
Ressources associées
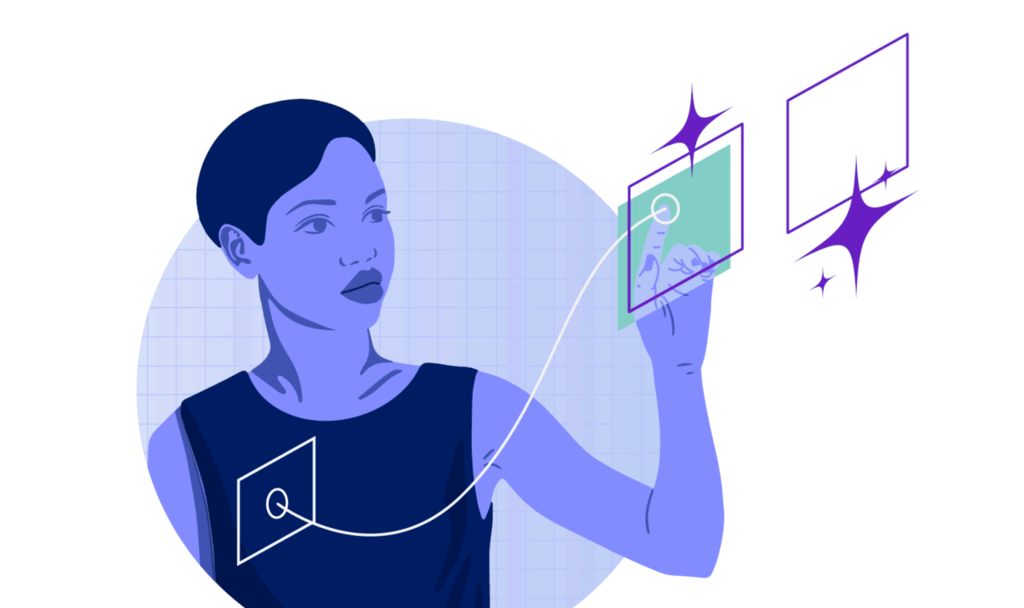
Exploitez la puissance de l'innovation par l'IA
Découvrez comment l'IA d'entreprise va contribuer à vos futurs résultats métier.
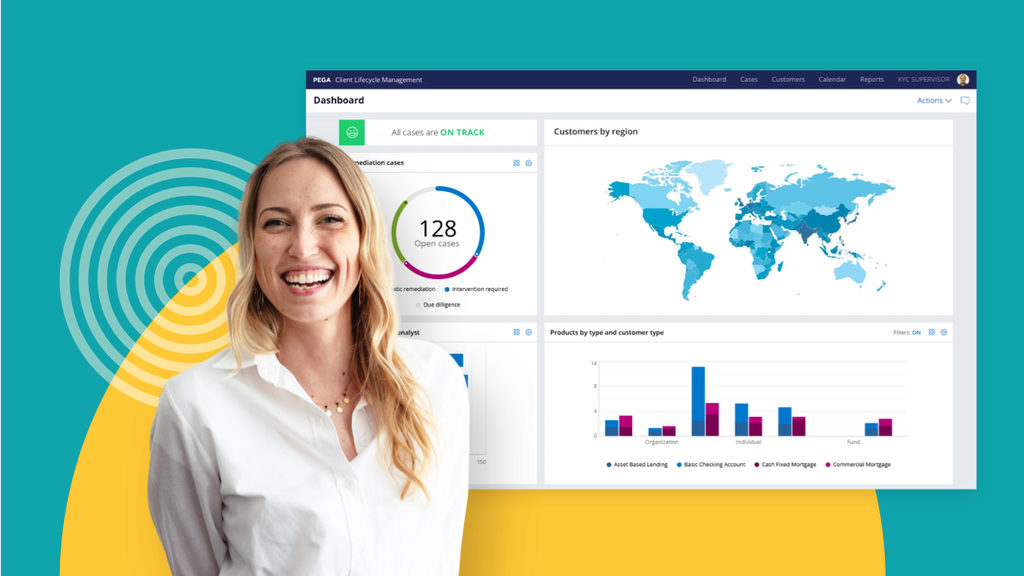
Pourquoi Pega ?
Notre puissant logiciel n'est pas notre seul atout.